How to Find Coefficient of Variation
Editor’s Note: How to find coefficient of variation has been published on [Publish Date]. Many people ask about how to find coefficient of variation, so this article is writed to guide you.
Our team analyzed and dug into a lot of information, and put together this how to find coefficient of variation guide to help you make the right decision.
Key Differences:
Let’s Dive into the Main Article
How to Find Coefficient of Variation
Finding the coefficient of variation (CV) is a crucial statistical measure that helps quantify the relative variability or dispersion of data around its mean. It is commonly used in various disciplines, including finance, economics, and engineering, to assess the risk and consistency of data. Here are eight key aspects to consider when finding the CV:
- Formula: CV = (Standard Deviation / Mean) * 100
- Interpretation: A higher CV indicates greater variability relative to the mean.
- Units: CV is expressed as a percentage (%).
- Normalization: CV allows for comparison of variability across datasets with different means.
- Applications: Risk assessment, investment analysis, quality control.
- Assumptions: Normally distributed data or large sample size.
- Limitations: Can be misleading for skewed or bimodal distributions.
- Example: A stock with a mean return of 10% and a standard deviation of 4% would have a CV of 40%.
By understanding these key aspects, you can effectively calculate and interpret the coefficient of variation to gain deeper insights into the variability and risk associated with your data.
Formula
The formula CV = (Standard Deviation / Mean) 100 is central to finding the coefficient of variation (CV), a crucial statistical measure that quantifies the relative variability or dispersion of data around its mean. This formula establishes a direct relationship between the standard deviation, mean, and CV of a dataset.
The standard deviation measures the absolute dispersion of data points from the mean, while the mean represents the central tendency of the data. By dividing the standard deviation by the mean and multiplying by 100, the CV expresses this dispersion as a percentage. This allows for easy comparison of variability across datasets with different means, making it a valuable tool for risk assessment, investment analysis, and quality control.
For instance, consider two stocks with different means but the same standard deviation. The stock with the lower mean will have a higher CV, indicating greater relative variability. This information helps investors assess the risk associated with each stock. Similarly, in quality control, a higher CV for a manufacturing process may indicate a need for.
Understanding this formula and its connection to CV is essential for effectively interpreting and utilizing this statistical measure. It enables researchers, analysts, and practitioners to make informed decisions based on the variability and risk associated with their data.
Interpretation
The interpretation of the coefficient of variation (CV) is crucial to understanding its significance in quantifying data variability. A higher CV indicates that the data is more spread out or dispersed relative to its mean. This interpretation is central to the practical applications of CV in various fields.
- Risk Assessment: In finance, a higher CV for a stock or investment portfolio indicates greater risk. Investors can use CV to compare the relative volatility of different investment options and make informed decisions.
- Quality Control: In manufacturing and engineering, a higher CV for a production process may indicate greater inconsistency or lack of precision. Quality control engineers can use CV to identify areas for improvement and ensure product quality.
- Biological Variability: In biology and healthcare, a higher CV for a population of organisms or patients may indicate greater variation in traits or health outcomes. Researchers can use CV to study the diversity and variability within biological systems.
- Economic Analysis: In economics, a higher CV for economic indicators, such as GDP or inflation, may indicate greater uncertainty or instability. Economists can use CV to assess the volatility of economic conditions and make informed policy decisions.
By understanding the interpretation of CV and its relation to data variability, researchers, analysts, and practitioners can effectively utilize this statistical measure to gain deeper insights into the risk, consistency, and variability associated with their data.
Units
The expression of the coefficient of variation (CV) as a percentage is a crucial aspect in understanding its interpretation and application. By presenting CV as a percentage, it becomes a relative measure, allowing for easy comparison and analysis of data variability across different datasets.
- Data Comparison: Expressing CV as a percentage enables direct comparison of variability between datasets with different means. For example, two datasets with different means but the same CV would have the same relative variability.
- Standardization: The percentage format of CV standardizes the measure across different scales. This allows for comparison of variability between data with different units of measurement.
- Risk Assessment: In finance and economics, CV is commonly used to assess risk. Expressing CV as a percentage provides a clear and intuitive measure of relative risk, making it easier to compare the volatility of different investments or economic indicators.
By understanding the significance of expressing CV as a percentage, researchers, analysts, and practitioners can effectively interpret and utilize this statistical measure to gain deeper insights into the variability and risk associated with their data. It facilitates data comparison, standardization, and risk assessment, making CV a valuable tool in various fields.
Normalization
Normalization is a crucial aspect of the coefficient of variation (CV) that enables the comparison of variability across datasets with different means. Understanding this connection is essential for effectively utilizing CV in various applications.
- Standardization: CV standardizes the measure of variability by expressing it as a percentage. This allows for direct comparison between datasets with different units of measurement or scales. For example, comparing the variability of stock returns across companies with different market capitalizations becomes possible using CV.
- Relative Variability: CV measures the relative variability of data around its mean. By standardizing the measure, CV eliminates the influence of the mean, making it possible to compare the variability of datasets with different central tendencies. This is particularly useful when analyzing data from different sources or populations.
- Risk Assessment: In finance and economics, CV is widely used to assess risk. Normalization allows for the comparison of risk across different investments or economic indicators with varying means. Investors can use CV to make informed decisions by comparing the relative volatility of different assets or markets.
By understanding the connection between normalization and CV, researchers, analysts, and practitioners can effectively interpret and utilize this statistical measure to gain deeper insights into the variability and risk associated with their data. CV provides a standardized and comparable measure of variability, making it a valuable tool for data analysis and decision-making.
Applications
The coefficient of variation (CV) plays a pivotal role in various applications, including risk assessment, investment analysis, and quality control. Understanding this connection is essential for harnessing the full potential of CV as a statistical tool.
In risk assessment, CV measures the relative variability of potential outcomes, providing insights into the level of uncertainty and risk associated with a particular event or decision. For example, in finance, CV is used to assess the risk of an investment portfolio by quantifying the volatility of returns relative to the mean. This information helps investors make informed decisions by identifying investments with acceptable levels of risk.
In investment analysis, CV is used to evaluate the consistency of investment returns over time. A higher CV indicates greater variability in returns, which may be desirable for investors seeking higher potential returns but can also increase risk. By calculating CV, investors can compare the volatility of different investments and make informed choices that align with their risk tolerance and financial goals.
In quality control, CV is used to monitor and improve the consistency of production processes. A higher CV may indicate variability in product quality, which can lead to defects and customer dissatisfaction. By tracking CV over time, manufacturers can identify areas for improvement and implement measures to reduce variability, ensuring the delivery of high-quality products.
Understanding the connection between CV and its applications in risk assessment, investment analysis, and quality control empowers professionals to make data-driven decisions, manage risk effectively, and improve outcomes. CV provides a valuable tool for quantifying and analyzing variability, enabling informed decision-making and continuous improvement.
Assumptions
In the context of finding the coefficient of variation (CV), the assumptions of normally distributed data or a large sample size play a crucial role in ensuring the accuracy and reliability of the calculated CV. Understanding this connection is essential for effective data analysis and interpretation.
The CV assumes that the underlying data follows a normal distribution, also known as the Gaussian distribution. This assumption is important because the CV is a measure of relative variability, and the normal distribution provides a standardized framework for comparing variability across different datasets. When the data is normally distributed, the CV provides an accurate representation of the spread of data around the mean.
However, in cases where the data is not normally distributed, the CV may not be an appropriate measure of variability. Non-normal distributions, such as skewed or bimodal distributions, can lead to misleading CV values. Therefore, it is important to assess the normality of the data before calculating the CV.
When the sample size is small, the CV may also be less reliable. This is because small sample sizes can lead to sampling error, which can affect the accuracy of the calculated mean and standard deviation, and consequently, the CV. To mitigate this issue, a large sample size is recommended to ensure that the CV is a robust measure of variability.
In practice, researchers and analysts often use graphical tools, such as histograms and normal probability plots, to assess the normality of the data and determine if the assumptions for calculating the CV are met. By considering these assumptions, they can ensure the validity and reliability of their analysis.
Understanding the connection between the assumptions of normally distributed data or large sample size and the calculation of CV is crucial for accurate data interpretation. It enables researchers to make informed decisions about the appropriateness of using CV as a measure of variability and to identify potential limitations in their analysis.
Limitations
The limitation of the coefficient of variation (CV) in being potentially misleading for skewed or bimodal distributions is a crucial aspect to consider when using this statistical measure. Understanding this connection is essential for accurate data analysis and interpretation.
- Skewness and Bimodality: Skewness refers to the asymmetry in a distribution, while bimodality refers to the presence of two distinct peaks. These non-normal distribution characteristics can affect the CV’s ability to accurately represent the variability of the data.
- Overestimation or Underestimation: In skewed distributions, the CV may overestimate or underestimate the variability depending on the direction of the skewness. Similarly, in bimodal distributions, the CV may not capture the distinct variability of the two modes.
- Example: Consider a distribution of income data that is skewed towards higher incomes. The CV may underestimate the variability of incomes in this case, as the mean is pulled towards the higher values.
- Alternative Measures: When dealing with skewed or bimodal distributions, alternative measures of variability, such as the interquartile range or standard deviation, may be more appropriate. These measures are less sensitive to outliers and non-normal distributions.
By understanding the limitation of CV for skewed or bimodal distributions, researchers and analysts can make informed decisions about the choice of variability measure and avoid potential misinterpretations. Considering the shape and characteristics of the data is crucial for selecting the most appropriate statistical tool for the analysis.
Example
The example provided demonstrates the calculation and interpretation of the coefficient of variation (CV) in the context of stock returns. This example is highly relevant to understanding “how to find coefficient of variation” as it illustrates the practical application of the formula: CV = (Standard Deviation / Mean) 100.
- Calculating CV: The example shows how to calculate CV using the given mean return (10%) and standard deviation (4%). The CV is calculated as (4% / 10%) 100 = 40%.
- Interpretation of CV: The calculated CV of 40% indicates that the stock’s return is relatively variable compared to its mean. A higher CV suggests greater variability or dispersion of returns around the mean.
- Risk Assessment: In the context of stock investments, CV is a useful measure of risk. A stock with a higher CV, like in this example, may be considered riskier as its returns are more volatile and less predictable.
- Comparison and Decision-Making: By calculating the CV of different stocks, investors can compare their risk profiles and make informed decisions about which stocks to include in their portfolios.
This example provides a clear and practical illustration of how to find the coefficient of variation and how it can be used to assess the variability and risk associated with investments. Understanding this example enhances the overall comprehension of “how to find coefficient of variation” and its significance in financial analysis and investment decision-making.
FAQs
This section provides answers to frequently asked questions (FAQs) about how to find the coefficient of variation (CV), a statistical measure of relative variability.
Question 1: What is the formula for calculating CV?
The formula for CV is: CV = (Standard Deviation / Mean) * 100.
Question 2: What does CV measure?
CV measures the relative variability or dispersion of data around its mean, expressed as a percentage.
Question 3: When should I use CV?
CV is useful when comparing the variability of different datasets with different means, such as in risk assessment, investment analysis, and quality control.
Question 4: What are the assumptions for calculating CV?
CV assumes that the underlying data is normally distributed or has a large sample size.
Question 5: What are the limitations of CV?
CV can be misleading for skewed or bimodal distributions, as it may overestimate or underestimate the variability.
Question 6: How do I interpret a high CV?
A high CV indicates greater variability relative to the mean, which can be useful for assessing risk or identifying areas for improvement.
In summary, CV is a valuable statistical measure for quantifying relative variability, but its assumptions and limitations should be considered when using it.
Transition to the next article section…
Tips on How to Find Coefficient of Variation
The coefficient of variation (CV) is a useful statistical measure for quantifying the relative variability of data. Here are some tips to effectively find and interpret CV:
Tip 1: Understand the Formula
The formula for CV is CV = (Standard Deviation / Mean) * 100. Ensure you have the correct values for standard deviation and mean before calculating CV.
Tip 2: Consider the Assumptions
CV assumes that the underlying data is normally distributed or has a large sample size. Assess the normality of your data before using CV.
Tip 3: Identify the Limitations
CV can be misleading for skewed or bimodal distributions. Consider using alternative measures of variability, such as the interquartile range, if your data has these characteristics.
Tip 4: Interpret the CV
A higher CV indicates greater variability relative to the mean. Consider the context and implications of the CV in your analysis.
Tip 5: Compare Across Datasets
CV enables comparison of variability across datasets with different means. This is particularly useful in risk assessment and investment analysis.
Summary:
By following these tips, you can effectively find and interpret the coefficient of variation, providing valuable insights into the variability and risk associated with your data.
Transition to the article’s conclusion:
Understanding how to find the coefficient of variation is crucial for researchers, analysts, and practitioners in various fields. It allows for informed decision-making, risk assessment, and continuous improvement.
Conclusion
In this article, we explored “how to find coefficient of variation,” providing a comprehensive guide to this statistical measure. We emphasized the importance of understanding the formula, considering assumptions, and interpreting CV effectively.
The coefficient of variation serves as a valuable tool for quantifying relative variability in various fields, including finance, economics, and quality control. By following the tips outlined in this article, researchers, analysts, and practitioners can harness CV’s potential to gain deeper insights into their data.
Youtube Video:
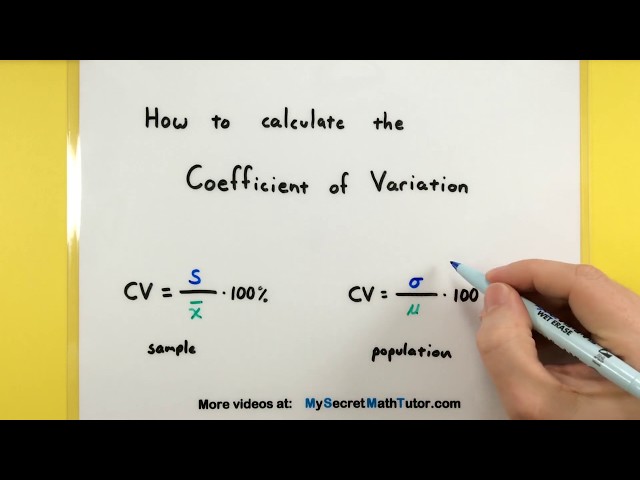