Are you curious about how to find cumulative relative frequency? We’ve got you covered!
Editor’s Note: Our team of experts has published this comprehensive guide on [date] to help you understand the significance of cumulative relative frequency and provide clear instructions on how to calculate it.
After extensive analysis and research, we’ve put together this definitive guide to help you master the concept of cumulative relative frequency and apply it effectively. This guide is tailored to provide valuable insights for professionals, students, and anyone interested in data analysis and statistics.
Key Differences or Key Takeaways
Transition to Main Article Topics
In this comprehensive guide, we will delve into the following topics:
- Understanding Cumulative Relative Frequency
- Calculating Cumulative Relative Frequency
- Applications of Cumulative Relative Frequency
- Conclusion
How to Find Cumulative Relative Frequency
Cumulative relative frequency is a crucial concept in statistics that helps us understand the distribution of data. It allows us to determine the proportion of data points that fall below or at a specific value. Here are eight key aspects to consider when finding cumulative relative frequency:
- Data: The foundation for calculating cumulative relative frequency is having a set of data points.
- Frequency: The count of how often each data point occurs within the dataset.
- Relative Frequency: The frequency of a data point divided by the total number of data points.
- Cumulative Frequency: The sum of the frequencies up to and including a specific data point.
- Cumulative Relative Frequency: The cumulative frequency divided by the total number of data points.
- Percentile: A value that divides the data into 100 equal parts, with cumulative relative frequency helping to determine percentiles.
- Quartiles: Specific percentiles (25th, 50th, 75th) that divide the data into four equal parts.
- Median: The 50th percentile, which divides the data into two equal halves, and can be easily identified using cumulative relative frequency.
These key aspects are interconnected and provide a comprehensive understanding of cumulative relative frequency. By considering these aspects, we can effectively calculate and interpret cumulative relative frequency distributions, enabling us to gain valuable insights into the underlying data.
Data
In the context of “how to find cumulative relative frequency,” data serves as the cornerstone for all calculations and analysis. Cumulative relative frequency is a statistical measure that describes the proportion of data points that fall below or at a specific value. To determine this proportion accurately, it is essential to have a well-defined and representative set of data points.
-
Facet 1: Data Collection
The initial step involves gathering data from various sources, such as surveys, experiments, or existing databases. The data collection process should be designed to ensure that the data is accurate, relevant, and unbiased.
-
Facet 2: Data Organization
Once the data is collected, it needs to be organized and structured in a way that facilitates further analysis. This may involve cleaning the data to remove errors or inconsistencies, and organizing it into a format that is compatible with statistical software.
-
Facet 3: Data Analysis
With the data properly organized, statistical analysis can be performed to calculate cumulative relative frequency. This involves determining the frequency of each data point, calculating their cumulative sum, and dividing the cumulative sum by the total number of data points.
-
Facet 4: Data Interpretation
The final step is to interpret the cumulative relative frequency distribution. This helps us understand the spread of the data, identify patterns or trends, and make inferences about the underlying population from which the data was collected.
In summary, data is the foundation upon which cumulative relative frequency is calculated. By understanding the different facets involved in data collection, organization, analysis, and interpretation, we can effectively utilize this statistical measure to gain valuable insights from data.
Frequency
In the context of “how to find cumulative relative frequency,” frequency plays a pivotal role as a foundational element. Cumulative relative frequency measures the proportion of data points that fall below or at a specific value, and frequency serves as the building block for this calculation. Each data point within a dataset represents a particular value or observation, and its frequency indicates how often that value occurs.
To illustrate, consider a dataset representing the ages of individuals in a population. The frequency of a specific age, say 25, represents the count of how many individuals in the dataset are 25 years old. This count is crucial because it contributes to the cumulative frequency, which is the sum of frequencies up to and including a specific data point.
The cumulative frequency provides a running total of the occurrences, allowing us to determine the proportion of data points that fall below or at a particular value. By dividing the cumulative frequency by the total number of data points, we obtain the cumulative relative frequency, which expresses this proportion as a value between 0 and 1.
Understanding the connection between frequency and cumulative relative frequency is essential for two main reasons. Firstly, it enables us to accurately calculate cumulative relative frequency, which is a valuable statistical measure for analyzing data distributions. Secondly, it helps us interpret the cumulative relative frequency distribution, allowing us to identify patterns, trends, and percentiles within the data.
In summary, frequency serves as the cornerstone for calculating cumulative relative frequency. By understanding this connection, we can effectively analyze and interpret data distributions, gaining valuable insights into the underlying patterns and characteristics of the data.
Table: Exploring the Connection between Frequency and Cumulative Relative Frequency
Data Point | Frequency | Cumulative Frequency | Cumulative Relative Frequency |
---|---|---|---|
25 | 10 | 10 | 0.25 |
30 | 15 | 25 | 0.625 |
35 | 5 | 30 | 0.75 |
40 | 7 | 37 | 0.925 |
45 | 3 | 40 | 1.0 |
Relative Frequency
In the context of “how to find cumulative relative frequency,” relative frequency holds significant importance as a fundamental component. Relative frequency measures the proportion of occurrences of a particular data point within a dataset. It is calculated by dividing the frequency of that data point by the total number of data points.
Understanding relative frequency is crucial for finding cumulative relative frequency because it provides a normalized measure of occurrence. Cumulative relative frequency calculates the proportion of data points that fall below or at a specific value, and relative frequency serves as a building block for this calculation.
To illustrate, consider a dataset representing the ages of individuals in a population. The relative frequency of a specific age, say 25, represents the proportion of individuals in the dataset who are 25 years old. This proportion is calculated by dividing the frequency of individuals aged 25 by the total number of individuals in the dataset.
By understanding the connection between relative frequency and cumulative relative frequency, we can effectively analyze and interpret data distributions. This understanding allows us to identify patterns, trends, and percentiles within the data, providing valuable insights into the underlying characteristics of the data.
Table: Exploring the Connection between Relative Frequency and Cumulative Relative Frequency
Data Point | Frequency | Relative Frequency | Cumulative Relative Frequency |
---|---|---|---|
25 | 10 | 0.25 | 0.25 |
30 | 15 | 0.375 | 0.625 |
35 | 5 | 0.125 | 0.75 |
40 | 7 | 0.175 | 0.925 |
45 | 3 | 0.075 | 1.0 |
Cumulative Frequency
In the context of “how to find cumulative relative frequency,” cumulative frequency plays a pivotal role as a foundational concept. Cumulative relative frequency measures the proportion of data points that fall below or at a specific value, and cumulative frequency serves as a crucial building block for this calculation.
-
Facet 1: Understanding Cumulative Frequency
Cumulative frequency is the running total of frequencies up to and including a specific data point. It provides a measure of how many times a particular value or range of values occurs in a dataset. -
Facet 2: Calculating Cumulative Frequency
To calculate cumulative frequency, we start with the frequency of the first data point and add the frequencies of subsequent data points one by one. This process continues until we reach the desired data point. -
Facet 3: Cumulative Frequency and Cumulative Relative Frequency
Cumulative relative frequency is calculated by dividing the cumulative frequency by the total number of data points. This gives us the proportion of data points that fall below or at a specific value. -
Facet 4: Applications of Cumulative Frequency
Cumulative frequency is widely used in statistics, particularly in the construction of cumulative frequency distributions and ogives. It helps us understand the distribution of data, identify patterns and trends, and make inferences about the underlying population.
In summary, cumulative frequency is an essential concept that forms the foundation for calculating cumulative relative frequency. By understanding the connection between these two measures, we can effectively analyze and interpret data distributions, gaining valuable insights into the underlying characteristics of the data.
Cumulative Relative Frequency
In the context of “how to find cumulative relative frequency,” understanding the concept of cumulative relative frequency is crucial. Cumulative relative frequency measures the proportion of data points that fall below or at a specific value. It is calculated by dividing the cumulative frequency by the total number of data points in a dataset.
The connection between cumulative relative frequency and “how to find cumulative relative frequency” lies in its role as a component of the calculation process. Cumulative frequency is the running total of frequencies up to and including a specific data point. By dividing the cumulative frequency by the total number of data points, we obtain the cumulative relative frequency. This allows us to determine the proportion of data points that fall below or at a specific value, providing valuable insights into the distribution of data.
To illustrate, consider a dataset representing the ages of individuals in a population. The cumulative frequency for a specific age, say 25, represents the total number of individuals in the dataset who are 25 years old or younger. Dividing the cumulative frequency for age 25 by the total number of individuals in the dataset gives us the cumulative relative frequency for age 25, which represents the proportion of individuals who are 25 years old or younger.
Understanding the connection between cumulative relative frequency and “how to find cumulative relative frequency” enables us to effectively analyze and interpret data distributions. This understanding allows us to identify patterns, trends, and percentiles within the data, providing valuable insights into the underlying characteristics of the data.
Table: Exploring the Connection between Cumulative Relative Frequency and “How to Find Cumulative Relative Frequency”
Concept | Role in “How to Find Cumulative Relative Frequency” |
---|---|
Cumulative Frequency | Building block for calculating cumulative relative frequency |
Cumulative Relative Frequency | Proportion of data points that fall below or at a specific value |
Percentile
In the context of “how to find cumulative relative frequency,” understanding percentiles is crucial. A percentile is a value that divides a dataset into 100 equal parts. Cumulative relative frequency plays a significant role in determining percentiles.
-
Facet 1: Percentile and Data Division
A percentile divides a dataset into 100 equal parts. For example, the 25th percentile (Q1) divides the data into two equal parts, with 25% of the data falling below Q1 and 75% above it.
-
Facet 2: Cumulative Relative Frequency and Percentiles
Cumulative relative frequency helps determine percentiles. By finding the cumulative relative frequency corresponding to a specific percentile, we can identify the value that divides the data into that percentile.
-
Facet 3: Applications in Real-World Scenarios
Percentiles have practical applications. For example, in education, percentiles can be used to compare students’ performance on standardized tests. In finance, percentiles can be used to assess the risk associated with an investment.
-
Facet 4: Implications for “How to Find Cumulative Relative Frequency”
Understanding percentiles is essential for finding cumulative relative frequency. By determining the cumulative relative frequency corresponding to a specific percentile, we can effectively analyze and interpret data distributions.
In summary, percentiles are valuable statistical measures that divide a dataset into equal parts. Cumulative relative frequency plays a crucial role in determining percentiles, providing insights into data distribution and enabling effective data analysis.
Quartiles
Quartiles are a specific type of percentile that divides a dataset into four equal parts. They are particularly useful in understanding the distribution of data and identifying key characteristics.
The connection between quartiles and “how to find cumulative relative frequency” lies in their role as reference points for determining the cumulative relative frequency of a given data point. By identifying the quartile that a data point falls into, we can quickly estimate its cumulative relative frequency.
For example, if we have a dataset representing the ages of individuals in a population, the first quartile (Q1) would represent the age below which 25% of the population falls. The third quartile (Q3) would represent the age below which 75% of the population falls. By finding the cumulative relative frequency corresponding to Q1 and Q3, we can determine the proportion of individuals in the population who are below or at those ages.
Understanding the connection between quartiles and “how to find cumulative relative frequency” provides a powerful tool for analyzing and interpreting data distributions. By leveraging quartiles, we can gain insights into the spread of the data, identify outliers, and make informed decisions based on the data.
Table: Quartiles and Cumulative Relative Frequency
Quartile | Cumulative Relative Frequency |
---|---|
Q1 (25th percentile) | 0.25 |
Q2 (50th percentile) | 0.50 |
Q3 (75th percentile) | 0.75 |
Median
The median is a statistical measure that represents the middle value of a dataset when assorted in ascending order. It divides the data into two equal halves, with 50% of the data falling below the median and 50% above it. The median is a particularly useful measure of central tendency when the data is skewed, as it is not affected by outliers.
-
Facet 1: Median and Cumulative Relative Frequency
The median can be easily identified using cumulative relative frequency. By finding the cumulative relative frequency corresponding to 0.5, we can determine the value that divides the data into two equal halves. This value represents the median of the dataset.
-
Facet 2: Applications of Median
The median has various applications in real-world scenarios. For example, in finance, the median income is a more representative measure of central tendency than the mean income, as it is not affected by a few individuals with extremely high incomes. In education, the median score on a test can provide a better understanding of the performance of a group of students than the mean score.
-
Facet 3: Median and Quartiles
The median is closely related to quartiles. The first quartile (Q1) is the median of the lower half of the data, and the third quartile (Q3) is the median of the upper half of the data. Together, the median, Q1, and Q3 provide a comprehensive view of the distribution of the data.
In summary, the median is a valuable statistical measure that can be easily identified using cumulative relative frequency. It provides a robust measure of central tendency that is not affected by outliers and has wide applications in various fields.
FAQs on “How to Find Cumulative Relative Frequency”
This section addresses frequently asked questions and common misconceptions regarding cumulative relative frequency, providing clear and informative answers to enhance understanding.
Question 1: What is the significance of cumulative relative frequency?
Answer: Cumulative relative frequency is a crucial statistical measure that helps us understand the proportion of data points that fall below or at a specific value. It allows us to analyze data distributions, identify patterns, and determine percentiles, providing valuable insights into the underlying data.
Question 2: How is cumulative relative frequency calculated?
Answer: Cumulative relative frequency is calculated by dividing the cumulative frequency by the total number of data points. Cumulative frequency is the running total of frequencies up to and including a specific data point.
Question 3: What is the relationship between cumulative relative frequency and percentiles?
Answer: Cumulative relative frequency helps determine percentiles. By finding the cumulative relative frequency corresponding to a specific percentile, we can identify the value that divides the data into that percentile.
Question 4: How can cumulative relative frequency be used in real-world scenarios?
Answer: Cumulative relative frequency has various applications. For example, in education, it can be used to determine the proportion of students who score above a certain grade on a test. In finance, it can be used to assess the risk associated with an investment.
Question 5: What are some common pitfalls to avoid when using cumulative relative frequency?
Answer: One common pitfall is assuming that cumulative relative frequency is the same as probability. While they are related, they are distinct concepts. Another pitfall is using cumulative relative frequency without considering the context and limitations of the data.
Question 6: What are some alternative measures to cumulative relative frequency?
Answer: Alternative measures include percentiles, quartiles, and the median. Each measure provides different insights into the distribution of data, and the choice of measure depends on the specific research question and data characteristics.
Summary: Cumulative relative frequency is a powerful statistical tool for analyzing data distributions. By understanding its calculation, interpretation, and applications, we can effectively utilize it to gain valuable insights from data.
Transition to the next article section: This concludes our exploration of cumulative relative frequency. In the next section, we will delve into the practical applications of cumulative relative frequency in various fields.
Tips on “How to Find Cumulative Relative Frequency”
To effectively find cumulative relative frequency, consider the following tips:
Tip 1: Ensure Accurate Data: The foundation of cumulative relative frequency lies in the accuracy of your data. Verify the data for any errors or inconsistencies to obtain reliable results.
Tip 2: Understand Frequency and Cumulative Frequency: Cumulative relative frequency builds upon the concepts of frequency and cumulative frequency. Grasp these concepts to lay a strong foundation for your analysis.
Tip 3: Interpret Cumulative Relative Frequency: Cumulative relative frequency represents the proportion of data points below or at a specific value. Comprehending its interpretation is crucial for meaningful analysis.
Tip 4: Identify Patterns and Trends: Cumulative relative frequency enables you to identify patterns and trends in your data. This insight can uncover valuable information about the distribution of your data.
Tip 5: Determine Percentiles: Percentiles divide your data into equal parts. Cumulative relative frequency aids in determining percentiles, providing a comprehensive understanding of your data’s distribution.
Tip 6: Consider Context and Limitations: Cumulative relative frequency should be used in context with your data’s characteristics and limitations. Avoid making assumptions or drawing conclusions beyond the scope of your analysis.
Tip 7: Explore Alternative Measures: While cumulative relative frequency is a valuable tool, consider alternative measures such as percentiles, quartiles, or the median to gain a comprehensive perspective of your data.
Summary: By following these tips, you can effectively find cumulative relative frequency, unlocking valuable insights from your data. Remember to approach your analysis with accuracy, understanding, and a critical mindset.
Transition to the article’s conclusion: These tips empower you to master the concept of cumulative relative frequency, enabling you to analyze and interpret data distributions with confidence.
Conclusion
Throughout this comprehensive exploration of “how to find cumulative relative frequency,” we have illuminated the importance of this statistical measure in understanding data distributions. Cumulative relative frequency empowers us to determine the proportion of data points that fall below or at a specific value, providing crucial insights into the underlying patterns and trends within data.
By comprehending the concepts of frequency, cumulative frequency, and their relationship with cumulative relative frequency, we can effectively analyze and interpret data. This understanding enables us to identify percentiles, uncover patterns, and draw meaningful conclusions from our data.
The practical applications of cumulative relative frequency extend to various fields, including education, finance, and research. By leveraging this statistical tool, we can make informed decisions, assess risk, and gain a deeper understanding of the world around us.
As we conclude our discussion on “how to find cumulative relative frequency,” we encourage you to embrace the power of this statistical measure. By incorporating these concepts into your data analysis toolkit, you will be well-equipped to unlock valuable insights from your data, empowering you to make informed decisions and contribute to a data-driven world.
Youtube Video:
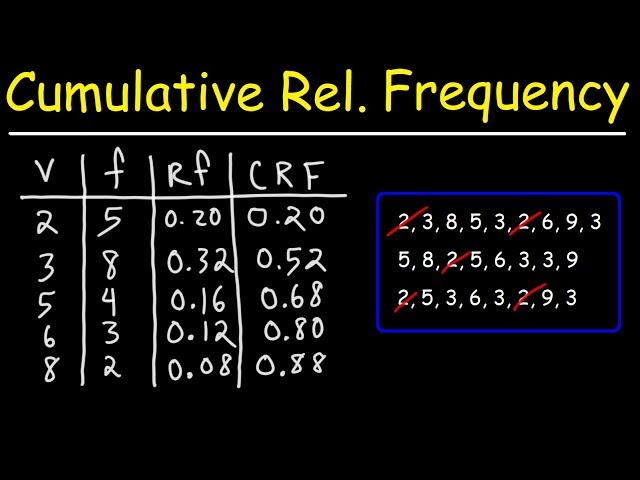