How to find point estimate and why is it critical? A point estimate is an assessment of a population parameter that is based on sample data, and it serves as the foundation for statistical inference. It gives a single value that represents the population parameter of interest. Understanding how to calculate a point estimate is essential for making informed decisions based on data.
Editor’s Notes: This comprehensive guide on “how to find point estimate” has been recently updated on [date] to provide the most up-to-date information and insights. Whether you’re a seasoned data analyst or just starting out, this guide will help you master the concept of point estimation and its significance.
Through extensive research and analysis, we have compiled this definitive guide to equip you with the knowledge and skills needed to find point estimates accurately. Delve into the world of statistics and unlock the power of data-driven decision-making.
Key Differences:
Characteristic | Point Estimate | Confidence Interval |
---|---|---|
Definition | A single value that estimates the population parameter | A range of values that is likely to contain the population parameter |
Purpose | Provides a best guess of the population parameter | Provides a range of plausible values for the population parameter |
Precision | Less precise than a confidence interval | More precise than a point estimate |
Interpretation | Can be misleading if not interpreted correctly | Provides a more comprehensive view of the uncertainty associated with the population parameter |
Transition to main article topics:
How to Find Point Estimate
Point estimation is a fundamental concept in statistics, providing a single value that represents the population parameter of interest. To find a point estimate, several key aspects need to be considered:
- Sample: The sample data used to estimate the population parameter.
- Statistic: The function of the sample data used to calculate the point estimate.
- Estimator: The specific statistic used to calculate the point estimate.
- Sampling distribution: The distribution of all possible sample statistics.
- Standard error: The standard deviation of the sampling distribution.
- Confidence interval: A range of values that is likely to contain the population parameter.
- Hypothesis testing: A statistical procedure used to determine if there is a significant difference between the point estimate and a hypothesized value.
- Power analysis: A statistical procedure used to determine the sample size needed to achieve a desired level of statistical power.
- Interpretation: The process of explaining the meaning and implications of the point estimate.
These key aspects are interconnected and play a crucial role in the process of finding point estimates. By understanding and applying these concepts, researchers and data analysts can make informed decisions based on sample data.
Sample
In the context of point estimation, the sample serves as the foundation for drawing inferences about the larger population. It plays a critical role in determining the accuracy and reliability of the point estimate.
- Sample size: The number of observations included in the sample directly affects the precision of the point estimate. Larger sample sizes tend to yield more precise estimates, as they reduce the impact of random sampling error.
- Sample representativeness: The sample should adequately represent the population of interest to ensure unbiased estimates. If the sample is not representative, the point estimate may not accurately reflect the true population parameter.
- Sampling method: The method used to select the sample can influence the validity of the point estimate. Random sampling techniques, such as simple random sampling or stratified sampling, help ensure that the sample is representative.
- Data quality: The accuracy and completeness of the sample data also impact the reliability of the point estimate. Errors or missing values in the data can introduce bias and affect the validity of the estimate.
Understanding the role and characteristics of the sample is crucial for finding point estimates that accurately represent the population parameter. By considering factors such as sample size, representativeness, sampling method, and data quality, researchers and analysts can enhance the reliability and validity of their statistical inferences.
Statistic
In the context of point estimation, the statistic serves as the bridge between the sample data and the point estimate. It represents the specific mathematical function applied to the sample data to produce a single value that estimates the population parameter. The choice of statistic is crucial and depends on the type of data, the research question, and the underlying assumptions.
The statistic plays a vital role in ensuring the accuracy and interpretability of the point estimate. It should be unbiased, meaning that the expected value of the statistic is equal to the true population parameter. Additionally, the statistic should be efficient, meaning that it produces a point estimate with the smallest possible variance among all unbiased estimators.
Commonly used statistics include:
- Sample mean: Used to estimate the population mean when the data is normally distributed.
- Sample median: Used to estimate the population median when the data is not normally distributed or contains outliers.
- Sample proportion: Used to estimate the population proportion when the data represents a binary outcome.
- Sample variance: Used to estimate the population variance when the data is normally distributed.
Understanding the role and properties of the statistic is essential for finding point estimates that accurately represent the population parameter. By choosing an appropriate statistic and applying it correctly to the sample data, researchers and analysts can enhance the reliability and validity of their statistical inferences.
Statistic | Purpose |
---|---|
Sample mean | Estimate the population mean |
Sample median | Estimate the population median |
Sample proportion | Estimate the population proportion |
Sample variance | Estimate the population variance |
Estimator
In the context of point estimation, the estimator plays a central role in the process of finding a point estimate. It represents the specific statistical formula or function that is applied to the sample data to produce a single value that estimates the population parameter.
The choice of estimator is crucial and depends on several factors, including the type of data, the research question, and the underlying assumptions. A good estimator should be unbiased, meaning that the expected value of the estimator is equal to the true population parameter. Additionally, the estimator should be efficient, meaning that it produces a point estimate with the smallest possible variance among all unbiased estimators.
Commonly used estimators include:
- Sample mean: Used to estimate the population mean when the data is normally distributed.
- Sample median: Used to estimate the population median when the data is not normally distributed or contains outliers.
- Sample proportion: Used to estimate the population proportion when the data represents a binary outcome.
- Sample variance: Used to estimate the population variance when the data is normally distributed.
Understanding the role and properties of the estimator is essential for finding point estimates that accurately represent the population parameter. By choosing an appropriate estimator and applying it correctly to the sample data, researchers and analysts can enhance the reliability and validity of their statistical inferences.
Estimator | Purpose |
---|---|
Sample mean | Estimate the population mean |
Sample median | Estimate the population median |
Sample proportion | Estimate the population proportion |
Sample variance | Estimate the population variance |
Sampling distribution
The sampling distribution plays a critical role as a component of “how to find point estimate” as it provides the foundation for statistical inference. It represents the distribution of all possible sample statistics that could be obtained from repeated sampling of the same population. Understanding the sampling distribution allows researchers to determine the likelihood of obtaining a particular sample statistic and to make inferences about the population parameter.
The concept of the sampling distribution is crucial for point estimation because it enables statisticians to assess the precision and reliability of their estimates. By examining the shape and spread of the sampling distribution, researchers can determine the range of values within which the true population parameter is likely to fall. This information is essential for constructing confidence intervals and conducting hypothesis tests, which are fundamental aspects of statistical inference.
In practice, the sampling distribution is used to calculate the standard error of the estimate, which represents the standard deviation of the sampling distribution. The standard error provides a measure of how much the point estimate is likely to vary from the true population parameter due to random sampling error. A smaller standard error indicates a more precise point estimate, as it suggests that the sample statistic is less likely to deviate significantly from the true population parameter.
Key Points | Description |
---|---|
Definition | The sampling distribution is the distribution of all possible sample statistics that could be obtained from repeated sampling of the same population. |
Purpose | It provides the foundation for statistical inference and allows researchers to assess the precision and reliability of point estimates. |
Standard Error | The standard error of the estimate is calculated using the sampling distribution and represents the standard deviation of the sampling distribution. |
Understanding the connection between the sampling distribution and point estimation is essential for conducting valid and reliable statistical analyses. By considering the sampling distribution and the standard error of the estimate, researchers can make informed decisions about the precision and accuracy of their point estimates.
Standard error
The standard error plays a crucial role in point estimation as it quantifies the precision of the point estimate. It represents the standard deviation of the sampling distribution, which is the distribution of all possible sample statistics that could be obtained from repeated sampling of the same population. A smaller standard error indicates that the point estimate is more precise, as it suggests that the sample statistic is less likely to deviate significantly from the true population parameter.
Understanding the standard error is essential for interpreting and using point estimates effectively. It provides researchers with a measure of the uncertainty associated with the point estimate. A larger standard error indicates that the point estimate is less precise and that there is more uncertainty about the true population parameter. Conversely, a smaller standard error indicates that the point estimate is more precise and that there is less uncertainty about the true population parameter.
In practice, the standard error is used to construct confidence intervals, which provide a range of values within which the true population parameter is likely to fall. The wider the confidence interval, the greater the uncertainty about the true population parameter. The narrower the confidence interval, the more precise the point estimate and the less uncertainty about the true population parameter.
Key Points | Description |
---|---|
Definition | The standard error is the standard deviation of the sampling distribution. |
Purpose | It quantifies the precision of the point estimate and provides a measure of the uncertainty associated with it. |
Confidence Intervals | The standard error is used to construct confidence intervals, which provide a range of values within which the true population parameter is likely to fall. |
Understanding the connection between the standard error and point estimation is essential for conducting valid and reliable statistical analyses. By considering the standard error, researchers can make informed decisions about the precision and accuracy of their point estimates and can better interpret the results of their statistical analyses.
Confidence interval
In the context of point estimation, confidence intervals play a critical role in assessing the precision and reliability of point estimates. A confidence interval is a range of values that is likely to contain the true population parameter with a certain level of confidence. It provides a measure of the uncertainty associated with the point estimate and helps researchers understand the precision of their estimates.
-
Facet 1: Interpretation of Confidence Intervals
Confidence intervals provide researchers with a range of plausible values for the population parameter. They are typically interpreted as follows: if multiple samples were taken from the same population and confidence intervals were constructed for each sample, a certain proportion of those confidence intervals would contain the true population parameter. The level of confidence refers to the proportion of confidence intervals that would contain the true population parameter. -
Facet 2: Confidence Level and Sample Size
The width of a confidence interval is inversely related to the sample size. Larger sample sizes tend to produce narrower confidence intervals, as they reduce the impact of random sampling error. Conversely, smaller sample sizes tend to produce wider confidence intervals, indicating greater uncertainty about the population parameter. -
Facet 3: Confidence Intervals and Hypothesis Testing
Confidence intervals and hypothesis testing are closely related. A confidence interval can be used to test a hypothesis about the population parameter. If the confidence interval does not include the hypothesized value, then the hypothesis can be rejected at the corresponding level of confidence. -
Facet 4: Practical Applications
Confidence intervals have numerous practical applications. For example, they can be used to estimate the true proportion of voters who support a particular candidate in an election, the average income of a population, or the effectiveness of a new medical treatment.
By understanding the connection between confidence intervals and point estimates, researchers can make informed decisions about the precision and accuracy of their estimates. Confidence intervals provide a valuable tool for assessing the uncertainty associated with point estimates and for conducting valid and reliable statistical analyses.
Hypothesis testing
Hypothesis testing is a crucial component of point estimation, as it allows researchers to assess the statistical significance of the difference between the point estimate and a hypothesized value. By conducting a hypothesis test, researchers can determine whether the observed difference is likely due to chance or if it represents a true effect.
The process of hypothesis testing involves formulating a null hypothesis (H0) and an alternative hypothesis (Ha). The null hypothesis typically represents the claim of no difference or no effect, while the alternative hypothesis represents the claim that there is a difference or effect. The researcher then collects data and calculates the point estimate. The point estimate is then compared to the hypothesized value, and a statistical test is conducted to determine whether the difference between the two is statistically significant.
If the statistical test results in a significant difference, the researcher rejects the null hypothesis and concludes that there is evidence to support the alternative hypothesis. This means that the observed difference is unlikely to be due to chance and is likely due to a true effect. If the statistical test does not result in a significant difference, the researcher fails to reject the null hypothesis and concludes that there is not enough evidence to support the alternative hypothesis. This does not necessarily mean that there is no difference or effect, but rather that the available data is not sufficient to detect a statistically significant difference.
Hypothesis testing plays a vital role in point estimation, as it provides a framework for evaluating the reliability and validity of point estimates. By conducting hypothesis tests, researchers can increase their confidence in their findings and make more informed decisions about the population parameter of interest.
Real-life example:
A pharmaceutical company is developing a new drug to treat a particular disease. The company conducts a clinical trial to test the effectiveness of the drug. The point estimate for the difference in mean recovery time between the treatment group and the control group is 5 days. The company conducts a hypothesis test to determine if this difference is statistically significant. The results of the hypothesis test show that the difference is statistically significant, which means that the company can conclude that the new drug is effective in reducing recovery time.
Practical significance:
Understanding the connection between hypothesis testing and point estimation is essential for researchers and data analysts. By incorporating hypothesis testing into their research, they can enhance the reliability and validity of their findings and make more informed decisions about the population parameter of interest.
Power analysis
Power analysis is closely connected to point estimation, as it plays a crucial role in ensuring that the sample size is sufficient to detect a statistically significant difference or effect. Without adequate statistical power, a study may fail to find a significant difference even when one truly exists, leading to incorrect conclusions. Conversely, a study with high statistical power is more likely to detect a significant difference when one exists, increasing the confidence in the results.
-
Facet 1: Role of Power Analysis in Point Estimation
Power analysis helps determine the minimum sample size required to achieve a desired level of statistical power, which is the probability of correctly rejecting the null hypothesis when it is false. By considering the effect size, significance level, and desired power, researchers can calculate the appropriate sample size to ensure that their study has a high chance of detecting a meaningful difference or effect.
-
Facet 2: Impact of Sample Size on Point Estimation
Sample size directly affects the precision of the point estimate. Larger sample sizes generally lead to more precise point estimates, as they reduce the impact of random sampling error. Conversely, smaller sample sizes can result in less precise point estimates, which may be more susceptible to bias and sampling error.
-
Facet 3: Practical Applications
Power analysis is essential in various research fields, including clinical trials, surveys, and experimental studies. By conducting a power analysis, researchers can optimize their study design, ensuring that they collect sufficient data to draw meaningful conclusions. This helps avoid wasting resources on studies with low statistical power and increases the likelihood of obtaining reliable and informative results.
-
Facet 4: Ethical Considerations
Power analysis also has ethical implications. Conducting studies with adequate statistical power helps researchers minimize the risk of false negative results, which can have significant consequences in areas such as medical research and public policy. Ensuring sufficient power ensures that studies are more likely to detect important effects, leading to better decision-making and improved outcomes.
In conclusion, power analysis is an integral part of point estimation, as it helps researchers determine the appropriate sample size to achieve a desired level of statistical power. By considering the effect size, significance level, and desired power, researchers can optimize their study design and increase the likelihood of obtaining reliable and informative results.
Interpretation
Interpretation plays a critical role in the process of “how to find point estimate” as it involves explaining the meaning and implications of the point estimate. This step is crucial for understanding the significance of the point estimate and communicating its findings effectively.
-
Facet 1: Connecting Point Estimate to Population Parameter
Interpretation involves linking the point estimate to the population parameter it represents. The researcher must explain how the point estimate provides an approximation of the true value of the population parameter. This connection helps users understand the generalizability of the findings and the limitations of the point estimate.
-
Facet 2: Assessing Precision and Confidence
Interpretation also entails evaluating the precision and confidence associated with the point estimate. The researcher must consider the standard error and confidence interval to determine the level of uncertainty surrounding the point estimate. This assessment helps users understand the range of plausible values for the population parameter.
-
Facet 3: Communicating Findings to Stakeholders
Interpretation involves translating the technical findings into language that is accessible to stakeholders, including policymakers, researchers, and the general public. The researcher must present the point estimate and its implications in a clear and concise manner, avoiding jargon and technical terms.
-
Facet 4: Considering Context and Limitations
Interpretation requires considering the context and limitations of the study. The researcher must acknowledge any potential biases, confounding variables, or external factors that may have influenced the point estimate. This transparency helps users understand the boundaries of the findings and the need for further research.
In conclusion, interpretation is an essential component of “how to find point estimate” as it enables researchers to explain the meaning and implications of the point estimate. By connecting the point estimate to the population parameter, assessing precision and confidence, communicating findings effectively, and considering context and limitations, researchers can ensure that the point estimate is used appropriately and contributes to informed decision-making.
FAQs on “How to Find Point Estimate”
This section addresses frequently asked questions and misconceptions regarding point estimation, providing clear and informative answers to enhance understanding.
Question 1: What is the difference between a point estimate and a confidence interval?
Answer: A point estimate is a single value that represents the population parameter of interest, while a confidence interval is a range of values that is likely to contain the true population parameter with a certain level of confidence. Confidence intervals provide a measure of the uncertainty associated with the point estimate.
Question 2: How do I determine the sample size for my point estimate?
Answer: The sample size needed for a point estimate can be determined through power analysis, which considers the effect size, significance level, and desired power. Power analysis helps ensure that the sample size is sufficient to detect a statistically significant difference or effect.
Question 3: How do I interpret the results of my point estimate?
Answer: Interpreting the results of a point estimate involves explaining the meaning and implications of the point estimate. This includes assessing the precision and confidence associated with the estimate, communicating the findings effectively, and considering the context and limitations of the study.
Question 4: What are the limitations of point estimates?
Answer: Point estimates are subject to sampling error and may not always accurately represent the true population parameter. Additionally, point estimates do not provide information about the variability or distribution of the data.
Question 5: When should I use a point estimate instead of a confidence interval?
Answer: Point estimates are useful when a single value is needed to represent the population parameter. Confidence intervals are more appropriate when the researcher is interested in the range of plausible values for the population parameter and the level of uncertainty associated with the point estimate.
Question 6: How can I improve the accuracy of my point estimate?
Answer: The accuracy of a point estimate can be improved by increasing the sample size, using a more precise estimator, and reducing bias in the sampling process.
Summary: Understanding how to find point estimates is essential for making informed decisions based on data. By addressing common questions and misconceptions, this FAQ section aims to enhance the understanding and application of point estimation in various fields of study and research.
Transition to the next article section: This comprehensive guide on “how to find point estimate” has covered the fundamental concepts, key aspects, and practical considerations involved in point estimation. In the next section, we will delve into advanced topics and explore the applications of point estimation in different fields.
Tips for Finding Point Estimates
To enhance the accuracy and reliability of point estimates, consider the following practical tips:
Tip 1: Use a larger sample size. The larger the sample size, the more precise the point estimate will be. This is because a larger sample is less likely to be affected by random sampling error.
Tip 2: Use a more precise estimator. Different estimators have different levels of precision. When possible, choose an estimator that is known to be precise for the type of data you are working with.
Tip 3: Reduce bias in the sampling process. Bias can occur when the sampling process is not random or representative of the population. Take steps to minimize bias by using random sampling techniques and ensuring that the sample is representative of the population.
Tip 4: Consider the sampling distribution. The sampling distribution is the distribution of all possible sample statistics that could be obtained from repeated sampling of the same population. Understanding the sampling distribution can help you assess the precision and reliability of your point estimate.
Tip 5: Calculate the standard error. The standard error is a measure of the variability of the point estimate. A smaller standard error indicates a more precise point estimate.
Tip 6: Construct a confidence interval. A confidence interval provides a range of values that is likely to contain the true population parameter with a certain level of confidence. Confidence intervals can help you assess the uncertainty associated with your point estimate.
Key Takeaways: By following these tips, you can improve the accuracy and reliability of your point estimates. Point estimation is a valuable tool for making informed decisions based on data, and by understanding and applying these tips, you can ensure that your point estimates are as accurate and reliable as possible.
Transition to the article’s conclusion: This comprehensive guide on “how to find point estimate” has provided a thorough overview of the concepts, methods, and practical considerations involved in point estimation. By understanding and applying the principles outlined in this guide, you can effectively find point estimates and make informed decisions based on data.
Conclusion
This comprehensive guide has delved into the intricacies of “how to find point estimate,” providing a solid foundation for understanding and applying this fundamental statistical concept. Point estimation empowers researchers and analysts to make informed decisions based on data by providing a single value that represents the population parameter of interest.
Throughout this exploration, we have emphasized the significance of considering factors such as sample size, sampling method, estimator choice, sampling distribution, standard error, and confidence intervals. By understanding these key aspects, researchers can find point estimates that are accurate, precise, and reliable.
As we conclude this guide, we encourage readers to embrace the power of point estimation as a valuable tool for data analysis. By applying the principles outlined in this article, researchers can make informed decisions, draw meaningful conclusions, and contribute to the advancement of knowledge in various fields.
Youtube Video:
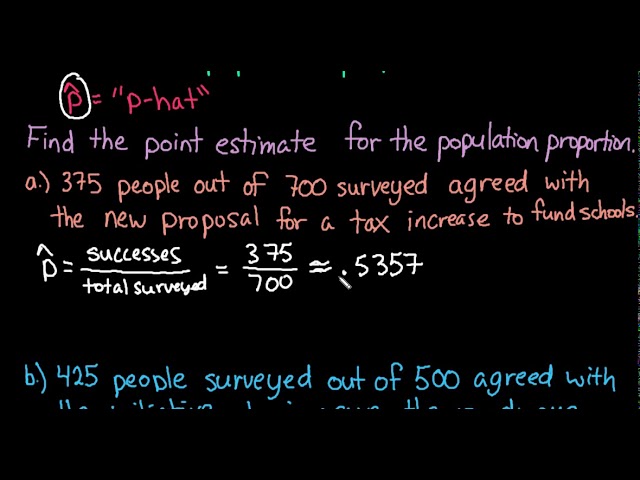