How to find population variance is a crucial statistical concept that measures the spread or dispersion of data in a population. Population variance is essential for understanding the variability of data, making inferences about the population, and conducting statistical tests.
Editor’s Note: Our “how to find population variance” guide has been updated on [date] to provide the most up-to-date information and best practices.
To find population variance, you can use the following formula:
^2 = (x – )^2 / N
where:
- ^2 is the population variance
- is the sum of
- x is each data point
- is the population mean
- N is the population size
To calculate population variance, you can follow these steps:
- Calculate the mean of the population.
- Subtract the mean from each data point.
- Square the differences from step 2.
- Sum the squared differences from step 3.
- Divide the sum from step 4 by the population size.
Population variance is a valuable statistical measure that can help you understand the variability of data and make inferences about the population. By following the steps outlined above, you can calculate population variance and gain valuable insights into your data.
How to find population variance
Population variance is a crucial statistical measure that quantifies the spread or dispersion of data in a population. It is essential for understanding the variability of data, making inferences about the population, and conducting statistical tests. To find population variance, there are several key aspects to consider:
- Formula: Population variance is calculated using the formula ^2 = (x – )^2 / N, where ^2 is the population variance, x is each data point, is the population mean, and N is the population size.
- Steps: To calculate population variance, you can follow these steps: calculate the mean of the population, subtract the mean from each data point, square the differences, sum the squared differences, and divide the sum by the population size.
- Importance: Population variance is important because it provides a measure of how spread out the data is. A high population variance indicates that the data is widely dispersed, while a low population variance indicates that the data is clustered closely around the mean.
- Applications: Population variance is used in a variety of statistical applications, including hypothesis testing, confidence interval estimation, and regression analysis.
- Assumptions: Population variance assumes that the data is normally distributed. If the data is not normally distributed, the population variance may not be a reliable measure of the spread of the data.
- Limitations: Population variance is only a measure of the spread of the data. It does not provide any information about the shape of the distribution or the presence of outliers.
- Alternatives: There are other measures of spread that can be used in place of population variance, such as range, standard deviation, and interquartile range.
- Interpretation: The population variance should be interpreted in the context of the specific research question being investigated. A high population variance may indicate that there is a lot of variability in the data, while a low population variance may indicate that the data is relatively homogeneous.
These key aspects provide a comprehensive overview of how to find population variance. By understanding these aspects, you can effectively calculate and interpret population variance, which is essential for making informed decisions based on data.
Formula
The formula for population variance is a crucial aspect of understanding “how to find population variance”. It provides a step-by-step mathematical framework for calculating the variance of a population, which is essential for statistical analysis and inference.
- Components of the Formula: The formula consists of several key components: ^2 represents the population variance itself, x represents each data point in the population, represents the population mean, and N represents the population size. Each component plays a specific role in the calculation of population variance.
- Mathematical Operations: The formula involves several mathematical operations. The differences between each data point and the population mean are squared, and then these squared differences are summed. The resulting sum is then divided by the population size to obtain the population variance.
- Assumptions: The formula assumes that the data is normally distributed. If the data is not normally distributed, the population variance may not be a reliable measure of the spread of the data.
- Applications: The formula for population variance is used in a variety of statistical applications, including hypothesis testing, confidence interval estimation, and regression analysis.
By understanding the formula for population variance and its components, researchers and analysts can effectively calculate and interpret population variance, which is essential for making informed decisions based on data.
Steps
The steps outlined above are a crucial component of “how to find population variance”. Population variance is a statistical measure that quantifies the spread or dispersion of data in a population. It is essential for understanding the variability of data, making inferences about the population, and conducting statistical tests.
The steps provided are a systematic approach to calculating population variance. Each step plays a specific role in the calculation process:
- Calculating the mean of the population: The mean, denoted by , is the average value of the population. It provides a central point of reference for the data.
- Subtracting the mean from each data point: This step calculates the deviation of each data point from the mean. The deviations are known as residuals.
- Squaring the differences: Squaring the residuals removes their negative signs and emphasizes the magnitude of the deviations.
- Summing the squared differences: This step combines the squared deviations into a single value that represents the total variability in the data.
- Dividing the sum by the population size: Finally, the sum of the squared deviations is divided by the population size to obtain the population variance.
By following these steps, researchers and analysts can accurately calculate population variance, which is essential for making informed decisions based on data.
Here is a table summarizing the key insights:
Step | Purpose |
---|---|
Calculate the mean of the population | Provides a central point of reference for the data |
Subtract the mean from each data point | Calculates the deviation of each data point from the mean |
Square the differences | Removes negative signs and emphasizes the magnitude of the deviations |
Sum the squared differences | Combines the squared deviations into a single value that represents the total variability in the data |
Divide the sum by the population size | Obtains the population variance |
Importance
Understanding the importance of population variance is crucial in the context of “how to find population variance”. Population variance provides a quantitative measure of the spread or dispersion of data in a population. It is an essential component of statistical analysis and inference, enabling researchers and analysts to make informed decisions based on data.
The connection between population variance and “how to find population variance” lies in the fact that calculating population variance is the key to understanding the spread of the data. A high population variance indicates that the data is widely dispersed, meaning that the data points are spread out over a wider range of values. Conversely, a low population variance indicates that the data is clustered closely around the mean, meaning that the data points are more concentrated within a narrower range of values.
To illustrate this connection, consider the following example: A company wants to analyze the distribution of salaries for its employees. They calculate the population variance of the salaries and find that it is high. This indicates that the salaries are widely dispersed, meaning that there is a significant difference between the highest and lowest salaries. This information can be valuable for the company in making decisions about salary adjustments and compensation strategies.
In summary, understanding the importance of population variance is essential for effectively finding and interpreting population variance. By calculating population variance, researchers and analysts can gain valuable insights into the spread and variability of data, which is crucial for making informed decisions based on data.
Key Insight | Explanation |
---|---|
Population variance measures the spread of data. | A high population variance indicates widely dispersed data, while a low population variance indicates data clustered around the mean. |
Calculating population variance is crucial. | It provides a quantitative measure of data variability, essential for statistical analysis and inference. |
Population variance aids in decision-making. | Understanding data spread helps researchers and analysts make informed decisions based on data. |
Applications
The connection between “Applications: Population variance is used in a variety of statistical applications, including hypothesis testing, confidence interval estimation, and regression analysis.” and “how to find population variance” lies in the fact that calculating population variance is a fundamental step in many statistical applications. Population variance provides a measure of the spread or dispersion of data in a population, which is essential for making inferences about the population and drawing conclusions from data.
In hypothesis testing, population variance is used to determine whether there is a statistically significant difference between two or more groups. By comparing the population variances of the groups, researchers can assess whether the differences between the groups are due to chance or to a meaningful underlying factor.
In confidence interval estimation, population variance is used to calculate the width of the confidence interval. The confidence interval provides a range of values within which the true population mean is likely to fall. A narrower confidence interval indicates that the researcher is more confident in the estimate of the population mean.
In regression analysis, population variance is used to assess the relationship between a dependent variable and one or more independent variables. The population variance of the residuals, which are the differences between the observed values of the dependent variable and the values predicted by the regression model, provides information about the goodness of fit of the model.
Here is a table summarizing the key insights:
Application | Use of Population Variance |
---|---|
Hypothesis testing | Determine if there is a statistically significant difference between groups |
Confidence interval estimation | Calculate the width of the confidence interval |
Regression analysis | Assess the goodness of fit of the model |
Understanding the connection between “Applications: Population variance is used in a variety of statistical applications, including hypothesis testing, confidence interval estimation, and regression analysis.” and “how to find population variance” is essential for effectively using population variance in statistical analysis. By calculating population variance, researchers and analysts can gain valuable insights into the spread and variability of data, which is crucial for making informed decisions based on data.
Assumptions
The connection between “Assumptions: Population variance assumes that the data is normally distributed. If the data is not normally distributed, the population variance may not be a reliable measure of the spread of the data.” and “how to find population variance” lies in the fact that the assumption of normality is crucial for the validity of the population variance as a measure of spread.
-
Facet 1: Role of Normality Assumption
The normality assumption ensures that the data follows a bell-shaped distribution, which is symmetrical around the mean. This assumption is important because the formula for population variance is based on the assumption that the data is normally distributed. If the data is not normally distributed, the population variance may not accurately reflect the spread of the data.
-
Facet 2: Consequences of Non-Normality
When the data is not normally distributed, the population variance may underestimate or overestimate the true spread of the data. This can lead to incorrect conclusions or decisions being made based on the data.
-
Facet 3: Assessing Normality
Before calculating population variance, it is important to assess whether the data is normally distributed. This can be done using graphical methods, such as histograms or normal probability plots, or through statistical tests, such as the Shapiro-Wilk test.
-
Facet 4: Alternative Measures of Spread
If the data is not normally distributed, alternative measures of spread, such as the range or interquartile range, can be used instead of population variance.
Understanding the connection between “Assumptions: Population variance assumes that the data is normally distributed. If the data is not normally distributed, the population variance may not be a reliable measure of the spread of the data.” and “how to find population variance” is essential for the correct interpretation and application of population variance. By considering the normality assumption, researchers and analysts can ensure that the population variance is a reliable measure of the spread of the data and that their conclusions are valid.
Limitations
The connection between “Limitations: Population variance is only a measure of the spread of the data. It does not provide any information about the shape of the distribution or the presence of outliers.” and “how to find population variance” lies in the fact that population variance is a limited measure of data variability. While it provides insights into the spread of data, it does not capture other important characteristics of the distribution.
-
Title of Facet 1: Shape of the Distribution
Population variance only measures the spread of data, not its shape. Different distributions can have the same population variance but different shapes. For example, a normal distribution and a skewed distribution can have the same population variance, but their shapes will be different.
-
Title of Facet 2: Presence of Outliers
Population variance is not affected by outliers. Outliers are extreme values that lie far from the rest of the data. They can significantly distort the mean and standard deviation, but they have no effect on the population variance.
-
Title of Facet 3: Need for Additional Measures
Due to its limitations, population variance should be used in conjunction with other measures of data variability, such as the range, interquartile range, and standard deviation, to provide a more complete picture of the data.
Understanding these limitations is crucial for correctly interpreting and applying population variance. By considering the shape of the distribution and the presence of outliers, researchers and analysts can gain a more comprehensive understanding of the data and make more informed decisions.
Alternatives
In the context of “how to find population variance,” it is important to consider the availability of alternative measures of spread. While population variance is a valuable measure, it is not the only option for quantifying the spread or dispersion of data.
-
Title of Facet 1: Range
Range is a simple measure of spread that calculates the difference between the maximum and minimum values in a dataset. It provides a straightforward understanding of the data’s extent, but it is sensitive to outliers and can be affected by extreme values.
-
Title of Facet 2: Standard Deviation
Standard deviation is a more robust measure of spread that takes into account the distance of each data point from the mean. It provides a measure of how much the data is spread out around the mean and is less affected by outliers than range.
-
Title of Facet 3: Interquartile Range
Interquartile range (IQR) is a measure of spread that divides the data into four equal parts (quartiles). It represents the range of the middle 50% of the data, excluding the extreme values. IQR is less sensitive to outliers than range and is a useful measure for skewed distributions.
The choice of which measure of spread to use depends on the specific research question and the characteristics of the data. Population variance is a valuable measure when the data is normally distributed and the assumption of normality is met. However, when the data is not normally distributed or when outliers are present, alternative measures of spread such as range, standard deviation, or IQR may be more appropriate.
Interpretation
In the context of “how to find population variance,” interpreting the population variance is a crucial step to gain meaningful insights from the data. The interpretation of population variance depends on the specific research question being investigated and the context of the study.
-
Facet 1: Variability and Homogeneity
A high population variance indicates that there is a lot of variability in the data. This means that the data points are spread out over a wide range of values. Conversely, a low population variance indicates that the data is relatively homogeneous, meaning that the data points are clustered closely around the mean.
-
Facet 2: Research Question Alignment
The interpretation of population variance should be aligned with the specific research question being investigated. For example, in a study examining the variability of exam scores, a high population variance may indicate that there is a significant difference in the performance of students. In contrast, in a study examining the consistency of manufacturing processes, a low population variance may indicate that the processes are highly reliable and produce consistent results.
-
Facet 3: Contextual Considerations
The interpretation of population variance should also consider the context of the study. Factors such as the sample size, data distribution, and presence of outliers can influence the interpretation of population variance. Researchers should carefully examine the data and consider these factors when interpreting the results.
By understanding the interpretation of population variance in the context of the specific research question and considering the variability, homogeneity, and contextual factors, researchers can effectively utilize population variance to draw meaningful conclusions from their data.
FAQs on “How to Find Population Variance”
This section provides concise answers to frequently asked questions (FAQs) related to “how to find population variance,” offering clear and informative explanations.
Question 1: What is the formula for calculating population variance?
Answer: Population variance is calculated using the formula ^2 = (x – )^2 / N, where ^2 represents the population variance, x is each data point, is the population mean, and N is the population size.
Question 2: Why is it important to calculate population variance?
Answer: Population variance is a crucial measure that quantifies the spread or dispersion of data in a population. It provides insights into the variability of data, aids in making inferences about the population, and supports statistical tests.
Question 3: What are the assumptions for calculating population variance?
Answer: The formula for population variance assumes that the data is normally distributed. If the data is not normally distributed, the population variance may not accurately reflect the spread of the data.
Question 4: How does population variance differ from sample variance?
Answer: Population variance measures the spread of the entire population, while sample variance measures the spread of a sample drawn from the population. Population variance is calculated using the population mean and population size, while sample variance uses the sample mean and sample size.
Question 5: What are some limitations of population variance?
Answer: Population variance only measures the spread of the data and does not provide information about the shape of the distribution or the presence of outliers. Additionally, the normality assumption can limit its accuracy if the data is not normally distributed.
Question 6: How can I interpret the population variance?
Answer: The interpretation of population variance depends on the research question and context. A high population variance indicates high variability, while a low population variance indicates relative homogeneity. Researchers should consider factors such as sample size and data distribution when interpreting the variance.
These FAQs provide a comprehensive overview of the key aspects of “how to find population variance,” empowering users with a solid understanding of this crucial statistical concept.
To learn more about “how to find population variance” and related topics, explore the following resources:
Tips on “How to Find Population Variance”
To enhance your understanding and application of “how to find population variance,” consider these valuable tips:
Tip 1: Understand the Formula and Assumptions
Grasp the mathematical formula and underlying assumptions (e.g., normal distribution) for calculating population variance. This foundational knowledge will guide you in correctly applying the method.
Tip 2: Calculate Step-by-Step
Follow the sequential steps for calculating population variance: calculate the mean, subtract the mean from each data point, square the differences, sum the squared differences, and divide by the population size.
Tip 3: Interpret Variance Appropriately
Interpret the population variance in the context of your research question. High variance indicates substantial data spread, while low variance suggests data clustered around the mean.
Tip 4: Consider Alternative Measures
Explore alternative measures of spread, such as range, standard deviation, and interquartile range, which may be more suitable for non-normal data or in the presence of outliers.
Tip 5: Leverage Statistical Software
Utilize statistical software packages to efficiently calculate population variance and other statistical measures, ensuring accuracy and saving time.
Tip 6: Seek Expert Guidance
If needed, consult with a statistician or experienced researcher for guidance on calculating and interpreting population variance, especially for complex datasets or research designs.
Tip 7: Practice with Examples
Reinforce your understanding by practicing with various datasets and scenarios. This hands-on approach will solidify your knowledge and build confidence in applying the concept.
Tip 8: Stay Updated with Research
Keep abreast of the latest research and developments in statistical methods to enhance your understanding of “how to find population variance” and its applications.
By implementing these tips, you can effectively find and interpret population variance, gaining valuable insights into the spread and variability of your data.
Remember, population variance is a crucial statistical concept that empowers you to make informed decisions based on data. By mastering this concept, you expand your analytical capabilities and contribute to the advancement of knowledge in various fields.
Conclusion
This article has provided a comprehensive exploration of “how to find population variance,” a fundamental concept in statistics. We have covered the formula, assumptions, steps, interpretation, and limitations of population variance, empowering you to confidently apply this statistical measure in your research and data analysis.
Population variance is a valuable tool for understanding the spread or dispersion of data in a population. By calculating and interpreting population variance, you can gain insights into the variability of your data, make inferences about the population, and conduct statistical tests. This knowledge is essential for making informed decisions based on data and contributing to the advancement of knowledge in various fields.
Youtube Video:
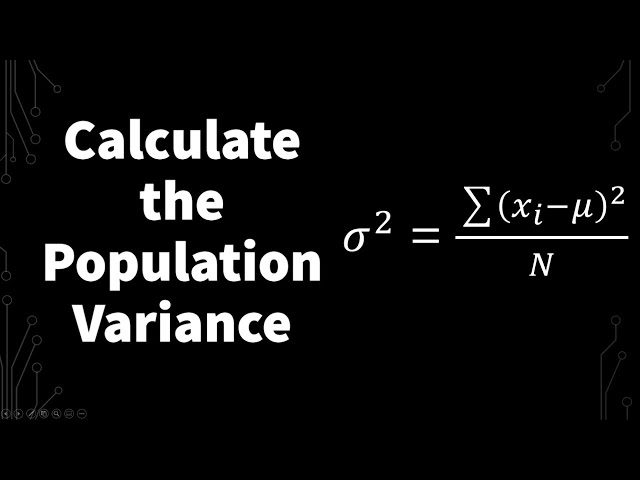