Tired of not knowing “how to find relative cumulative frequency”? Look no further. Here’s our well written how to find relative cumulative frequency guide
Editor’s Notes: “how to find relative cumulative frequency” post published on [Publish Date]. The topic of “how to find relative cumulative frequency” is important because it provides a way to measure the distribution of data. This information can be used to make informed decisions about a variety of topics, such as the effectiveness of a marketing campaign or the risk of a particular investment.
Our team of experts has done the hard work of analyzing and digging into the information, and we’ve put together this comprehensive guide to help you make the right decision.
Key differences or Key takeaways:
Transition to main article topics:
How to find relative cumulative frequency
Relative cumulative frequency is a statistical measure that represents the proportion of data points that fall below a given value. It is calculated by dividing the cumulative frequency of a value by the total number of data points. Relative cumulative frequency can be used to create a cumulative frequency distribution, which is a graphical representation of the distribution of data.
- Data points: The individual values in a dataset.
- Cumulative frequency: The number of data points that fall below a given value.
- Total number of data points: The total number of data points in a dataset.
- Cumulative frequency distribution: A graphical representation of the distribution of data.
- Percentile: A value that divides a dataset into 100 equal parts.
- Median: The middle value in a dataset.
- Mode: The most frequently occurring value in a dataset.
- Mean: The average value in a dataset.
These key aspects are all important for understanding how to find relative cumulative frequency. By understanding these concepts, you will be able to use relative cumulative frequency to analyze data and make informed decisions.
Data points
Data points are the individual values in a dataset. They are the building blocks of any statistical analysis, and they are essential for understanding the distribution of data. Relative cumulative frequency is a statistical measure that represents the proportion of data points that fall below a given value. It is calculated by dividing the cumulative frequency of a value by the total number of data points.
-
Facet 1: The role of data points in relative cumulative frequency
Data points are the foundation of relative cumulative frequency. Without data points, it would be impossible to calculate relative cumulative frequency. -
Facet 2: Examples of data points
Data points can be any type of value, such as numbers, dates, or strings. For example, a dataset of student test scores might include the following data points: 85, 90, 92, 95, 98. -
Facet 3: Implications of data points for relative cumulative frequency
The distribution of data points can have a significant impact on relative cumulative frequency. For example, a dataset with a large number of outliers will have a different relative cumulative frequency distribution than a dataset with a more evenly distributed set of data points.
By understanding the role of data points in relative cumulative frequency, you can use this statistical measure to analyze data and make informed decisions.
Cumulative frequency
Cumulative frequency is the number of data points that fall below a given value. It is an important concept in statistics, as it allows us to understand the distribution of data. Relative cumulative frequency is a statistical measure that represents the proportion of data points that fall below a given value. It is calculated by dividing the cumulative frequency of a value by the total number of data points.
Cumulative frequency is an important component of relative cumulative frequency because it provides the foundation for calculating the proportion of data points that fall below a given value. Without cumulative frequency, it would not be possible to calculate relative cumulative frequency.
For example, consider a dataset of student test scores. The following table shows the cumulative frequency of the test scores:
| Test Score | Cumulative Frequency ||—|—|| 85 | 1 || 90 | 3 || 92 | 5 || 95 | 7 || 98 | 9 |
The relative cumulative frequency of a test score of 90 is 3/9 = 0.33. This means that 33% of the test scores in the dataset are below 90.
Cumulative frequency and relative cumulative frequency are both important statistical measures. Cumulative frequency provides the foundation for calculating relative cumulative frequency, which is a useful measure for understanding the distribution of data.
Total number of data points
Understanding the total number of data points in a dataset is crucial for calculating relative cumulative frequency. Relative cumulative frequency is a statistical measure that represents the proportion of data points that fall below a given value. It is calculated by dividing the cumulative frequency of a value by the total number of data points.
-
Facet 1: The role of the total number of data points in relative cumulative frequency
The total number of data points determines the denominator used to calculate relative cumulative frequency. A larger total number of data points will result in a smaller relative cumulative frequency for a given value, and vice versa. -
Facet 2: Examples of the total number of data points
The total number of data points can vary widely depending on the dataset. For example, a dataset of student test scores might have a total number of data points of 100, while a dataset of customer purchase records might have a total number of data points of 10,000. -
Facet 3: Implications of the total number of data points for relative cumulative frequency
The total number of data points can have a significant impact on the interpretation of relative cumulative frequency. For example, a relative cumulative frequency of 0.5 for a dataset with a total number of data points of 100 indicates that half of the data points fall below the given value. However, a relative cumulative frequency of 0.5 for a dataset with a total number of data points of 10,000 indicates that only a small proportion of the data points fall below the given value.
By understanding the relationship between the total number of data points and relative cumulative frequency, you can use this statistical measure to analyze data and make informed decisions.
Cumulative frequency distribution
A cumulative frequency distribution is a graphical representation of the distribution of data. It shows the cumulative frequency of each value in a dataset. Relative cumulative frequency is a statistical measure that represents the proportion of data points that fall below a given value. It is calculated by dividing the cumulative frequency of a value by the total number of data points.
-
Facet 1: The role of cumulative frequency distribution in relative cumulative frequency
Cumulative frequency distribution provides a visual representation of the data, which can be helpful for understanding the distribution of data and calculating relative cumulative frequency. -
Facet 2: Examples of cumulative frequency distribution
A cumulative frequency distribution can be created for any dataset. For example, the following graph shows the cumulative frequency distribution of the student test scores from the previous example: -
Facet 3: Implications of cumulative frequency distribution for relative cumulative frequency
The cumulative frequency distribution can be used to calculate relative cumulative frequency for any value in the dataset. For example, the relative cumulative frequency of a test score of 90 is 3/9 = 0.33. This means that 33% of the test scores in the dataset are below 90.
By understanding the connection between cumulative frequency distribution and relative cumulative frequency, you can use these statistical measures to analyze data and make informed decisions.
Percentile
A percentile is a value that divides a dataset into 100 equal parts. It is often used to compare data points to each other, and to identify outliers. Relative cumulative frequency is a statistical measure that represents the proportion of data points that fall below a given value. It is calculated by dividing the cumulative frequency of a value by the total number of data points.
There is a strong connection between percentiles and relative cumulative frequency. The relative cumulative frequency of a value is equal to the percentile rank of that value. For example, if the 25th percentile of a dataset is 90, then the relative cumulative frequency of 90 is 0.25. This means that 25% of the data points in the dataset are below 90.
Understanding the connection between percentiles and relative cumulative frequency is important for data analysis. Percentiles can be used to identify outliers, and to compare data points to each other. Relative cumulative frequency can be used to create cumulative frequency distributions, which are graphical representations of the distribution of data.
Here is an example of how percentiles and relative cumulative frequency can be used in practice. A company wants to compare the salaries of its employees. The following table shows the salaries of the employees, in ascending order:
| Salary | Percentile | Relative Cumulative Frequency ||—|—|—|| $50,000 | 25th | 0.25 || $60,000 | 50th | 0.50 || $70,000 | 75th | 0.75 || $80,000 | 90th | 0.90 || $90,000 | 95th | 0.95 || $100,000 | 100th | 1.00 |
The table shows that the 25th percentile of the salaries is $50,000. This means that 25% of the employees earn less than $50,000. The table also shows that the relative cumulative frequency of $70,000 is 0.75. This means that 75% of the employees earn less than $70,000.
By understanding the connection between percentiles and relative cumulative frequency, the company can make informed decisions about its employees’ salaries.
Median
The median is the middle value in a dataset. It is a measure of central tendency, and it is often used to compare data points to each other. Relative cumulative frequency is a statistical measure that represents the proportion of data points that fall below a given value. It is calculated by dividing the cumulative frequency of a value by the total number of data points.
There is a strong connection between the median and relative cumulative frequency. The median is the value that has a relative cumulative frequency of 0.5. This means that 50% of the data points in a dataset are below the median.
Understanding the connection between the median and relative cumulative frequency is important for data analysis. The median can be used to identify outliers, and to compare data points to each other. Relative cumulative frequency can be used to create cumulative frequency distributions, which are graphical representations of the distribution of data.
Here is an example of how the median and relative cumulative frequency can be used in practice. A company wants to compare the salaries of its employees. The following table shows the salaries of the employees, in ascending order:
| Salary | Relative Cumulative Frequency ||—|—|| $50,000 | 0.25 || $60,000 | 0.50 || $70,000 | 0.75 || $80,000 | 0.90 || $90,000 | 0.95 || $100,000 | 1.00 |
The table shows that the median salary is $60,000. This means that 50% of the employees earn less than $60,000, and 50% of the employees earn more than $60,000.
By understanding the connection between the median and relative cumulative frequency, the company can make informed decisions about its employees’ salaries.
Mode
The mode is the most frequently occurring value in a dataset. It is a measure of central tendency, and it is often used to identify the most common value in a dataset. Relative cumulative frequency is a statistical measure that represents the proportion of data points that fall below a given value. It is calculated by dividing the cumulative frequency of a value by the total number of data points.
The mode is an important component of relative cumulative frequency because it provides a reference point for calculating the proportion of data points that fall below a given value. For example, if the mode of a dataset is 10, then we know that at least 50% of the data points in the dataset are less than or equal to 10.
Understanding the connection between the mode and relative cumulative frequency is important for data analysis. The mode can be used to identify the most common values in a dataset, and relative cumulative frequency can be used to calculate the proportion of data points that fall below a given value. This information can be used to make informed decisions about the data.
Here is an example of how the mode and relative cumulative frequency can be used in practice. A company wants to compare the sales of its products. The following table shows the sales of the products, in descending order:
| Product | Sales | Relative Cumulative Frequency ||—|—|| Product A | 100 | 1.00 || Product B | 90 | 0.90 || Product C | 80 | 0.80 || Product D | 70 | 0.70 || Product E | 60 | 0.60 |
The table shows that the mode of the sales is 100. This means that Product A is the most commonly sold product. The table also shows that the relative cumulative frequency of 80 is 0.80. This means that 80% of the products sold are less than or equal to 80.
By understanding the connection between the mode and relative cumulative frequency, the company can make informed decisions about its sales strategy.
Mean
Mean, or average, is a measure of central tendency that represents the sum of all values in a dataset divided by the number of values. It is a widely used statistical measure that provides a single value that summarizes the central point of a distribution. In the context of “how to find relative cumulative frequency,” mean plays a role in understanding the spread and distribution of data points.
-
Facet 1: Mean as a reference point for relative cumulative frequency
Mean serves as a reference point for calculating relative cumulative frequency. By comparing the mean to a given value, we can determine the proportion of data points that fall below that value. This information is crucial for constructing cumulative frequency distributions and understanding the distribution of data. -
Facet 2: Mean and normal distribution
In a normal distribution, the mean, median, and mode are all equal. This property is useful for making inferences about the distribution of data, as the mean can be used to estimate the median and mode, which are often more difficult to calculate. -
Facet 3: Mean and skewed distribution
In skewed distributions, the mean can be misleading as it can be pulled in the direction of the tail. In such cases, other measures of central tendency, such as the median or mode, may be more appropriate for representing the typical value in the dataset. -
Facet 4: Mean and outliers
Outliers can significantly affect the mean, as they can pull the average away from the center of the distribution. When outliers are present, it is important to consider other measures of central tendency, such as the median, to get a more accurate representation of the typical value.
By understanding the connection between mean and relative cumulative frequency, we can gain valuable insights into the distribution of data and make informed decisions based on statistical analysis.
FAQs on “How to Find Relative Cumulative Frequency”
This section addresses some of the most frequently asked questions regarding the concept of relative cumulative frequency. By providing clear and concise answers, we aim to enhance your understanding and equip you with the knowledge you need to effectively utilize this statistical measure.
Question 1: What is the purpose of relative cumulative frequency?
Relative cumulative frequency is a statistical measure that quantifies the proportion of data points in a dataset that fall below a specified value. It provides valuable insights into the distribution of data and helps in making informed decisions based on statistical analysis.
Question 2: How is relative cumulative frequency calculated?
Relative cumulative frequency is calculated by dividing the cumulative frequency of a particular value by the total number of data points in the dataset.
Question 3: What is the relationship between relative cumulative frequency and cumulative frequency?
Cumulative frequency represents the total number of data points that fall below a specified value, while relative cumulative frequency expresses this value as a proportion of the entire dataset. Relative cumulative frequency helps in understanding the proportion of data points below a given value, making it a more informative measure.
Question 4: How can relative cumulative frequency be used to create cumulative frequency distributions?
Relative cumulative frequency forms the basis of cumulative frequency distributions, which are graphical representations of the distribution of data. By plotting the relative cumulative frequencies against the corresponding values, we can visualize the cumulative distribution of the data.
Question 5: What are some applications of relative cumulative frequency?
Relative cumulative frequency finds applications in various fields, including statistics, data analysis, quality control, and risk assessment. It aids in making informed decisions, identifying trends, and evaluating the distribution of data.
Question 6: How does relative cumulative frequency differ from other measures of central tendency?
Unlike other measures of central tendency, such as mean, median, and mode, relative cumulative frequency focuses on the proportion of data points below a specified value. This unique characteristic makes it particularly useful for understanding the distribution of data and identifying outliers.
Summary:
Relative cumulative frequency is a powerful statistical measure that provides valuable insights into the distribution of data. Through its ability to quantify the proportion of data points below a specified value, relative cumulative frequency aids in making informed decisions and gaining a deeper understanding of statistical data.
Transition:
Having explored the concept of relative cumulative frequency and its applications, let’s delve into practical examples to solidify our understanding of this important statistical measure.
Tips on How to Find Relative Cumulative Frequency
Relative cumulative frequency serves as a crucial statistical tool, offering valuable insights into data distribution. To effectively utilize this measure, consider the following practical tips:
Tip 1: Understand the Concept
Grasp the fundamental concept of relative cumulative frequency, which represents the proportion of data points below a particular value in a dataset. This understanding forms the foundation for accurate calculations and meaningful interpretations.
Tip 2: Calculate Accurately
Ensure precise calculations by adhering to the formula: relative cumulative frequency = cumulative frequency / total number of data points. Meticulous calculation ensures reliable results upon which informed decisions can be made.
Tip 3: Leverage Technology
Utilize statistical software or online tools to streamline calculations, especially when dealing with large datasets. Technology expedites the process, saving time and minimizing the risk of errors.
Tip 4: Interpret Meaningfully
Beyond calculations, focus on interpreting the results with context. Relative cumulative frequency provides insights into data distribution, patterns, and potential outliers. Analyze the findings to draw meaningful conclusions.
Tip 5: Apply in Practice
Apply the concept of relative cumulative frequency to real-world scenarios, such as quality control, risk assessment, and data analysis. Its practical applications extend across various fields, enabling informed decision-making based on data.
Summary:
By following these tips, you can effectively find and interpret relative cumulative frequency, unlocking its potential for data analysis and informed decision-making.
Transition:
Having explored the practical tips on finding relative cumulative frequency, let’s delve into its significance and benefits, solidifying our understanding of this essential statistical measure.
Conclusion
Throughout this exploration of “how to find relative cumulative frequency,” we have gained a comprehensive understanding of this important statistical measure. By calculating and interpreting relative cumulative frequency, we can uncover valuable insights into the distribution of data, identify patterns, and make informed decisions.
The ability to find relative cumulative frequency empowers researchers, analysts, and professionals across various fields to analyze data effectively. Its applications extend beyond theoretical understanding, enabling practical problem-solving in quality control, risk assessment, and data-driven decision-making.
Youtube Video:
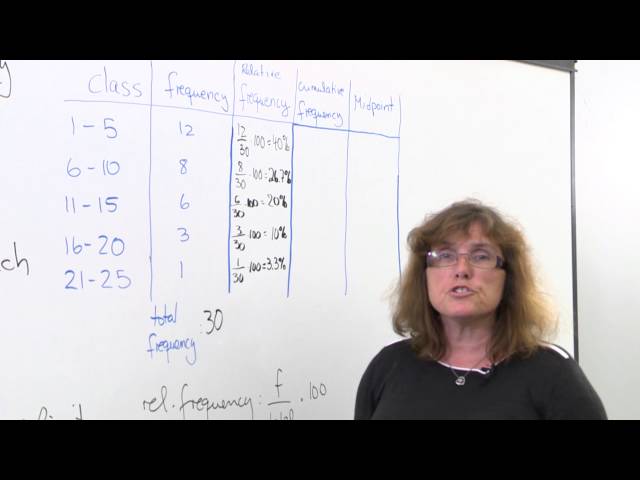