Looking to uncover the secrets of finding the lower quartile? Look no further! We’ve got you covered with our ultimate guide that unveils the mysteries behind this statistical measure.
Editor’s Notes: Our comprehensive guide on “how to find the lower quartile” published on [date], provides invaluable insights into this crucial concept. Understanding the lower quartile empowers you to make informed decisions and gain a deeper understanding of data analysis.
Through meticulous analysis and extensive research, we’ve crafted this definitive guide to help you master the art of finding the lower quartile. Whether you’re a seasoned statistician or just starting your journey into data exploration, this guide will equip you with the knowledge and skills you need to conquer this statistical challenge.
Key Differences: Understanding the Lower Quartile
Lower Quartile | |
---|---|
Definition | The value that separates the bottom 25% of data from the top 75%. |
Formula | Q1 = (n+1)/4th value in the ordered dataset |
Interpretation | Indicates the value below which 25% of the data lies. |
Dive into the Details: A Step-by-Step Guide
How to Find the Lower Quartile
Unveiling the lower quartile, a crucial statistical measure, requires a comprehensive understanding of its key aspects. Here’s a concise exploration of nine essential elements:
- Definition: Bottom 25% of data.
- Formula: (n+1)/4th value in ordered data.
- Interpretation: 25% of data lies below this value.
- Significance: Indicates data distribution and variability.
- Applications: Data analysis, quality control, risk assessment.
- Median Relationship: Lower quartile is below the median.
- Interquartile Range: Distance between lower and upper quartiles.
- Example: In a dataset of exam scores, the lower quartile represents the score below which 25% of students scored.
- Relevance: Understanding the lower quartile provides valuable insights into data distribution and patterns.
These aspects collectively provide a solid foundation for comprehending the lower quartile. By delving into these elements, we gain a deeper appreciation for the significance and applications of this statistical measure. Whether analyzing data for research, making informed decisions, or simply understanding the world around us, the lower quartile plays a crucial role in our exploration and interpretation of data.
Definition
Comprehending the definition of the lower quartile as “the value that separates the bottom 25% of data from the top 75%” is crucial for understanding how to find it. This definition establishes the lower quartile’s fundamental role in describing data distribution and variability.
To calculate the lower quartile, we must first order the data from smallest to largest. The lower quartile is then determined by identifying the value that marks the boundary between the bottom 25% and the top 75% of the data. This value effectively divides the data into four equal parts, providing insights into the data’s central tendency and spread.
For instance, consider a dataset representing the exam scores of a class. The lower quartile of this dataset would represent the score below which 25% of the students scored. This information is valuable for educators as it helps them identify areas where students may need additional support or targeted interventions.
Overall, understanding the definition of the lower quartile as the bottom 25% of data is essential for accurately calculating this statistical measure. It provides the foundation for data analysis, enabling researchers and practitioners to make informed decisions based on a comprehensive understanding of data distribution and variability.
Lower Quartile | |
---|---|
Definition | The value that separates the bottom 25% of data from the top 75%. |
Formula | (n+1)/4th value in the ordered dataset |
Interpretation | Indicates the value below which 25% of the data lies. |
Formula
The formula (n+1)/4th value in ordered data serves as a cornerstone for calculating the lower quartile, offering a systematic approach to identify the boundary between the bottom 25% and top 75% of data. To fully grasp the significance of this formula, let’s explore its components and their implications:
- Total Number of Data Points (n): The formula begins with ‘n,’ representing the total number of data points in the dataset. Understanding the size of the dataset is crucial for correctly determining the position of the lower quartile.
- Ordered Data: Before applying the formula, the data must be arranged in ascending order, from smallest to largest. This ordering ensures that the lower quartile accurately reflects the distribution of data.
- (n+1)/4: The formula (n+1)/4 calculates a specific position within the ordered dataset. This position represents the boundary between the bottom 25% and top 75% of data.
- 4th Value: Once the position is determined, the 4th value in the ordered dataset corresponds to the lower quartile. This value effectively divides the data into four equal parts, providing insights into the central tendency and variability.
In summary, the formula (n+1)/4th value in ordered data provides a structured method for finding the lower quartile. By understanding the components of this formula and their implications, we gain a deeper appreciation for its role in statistical analysis and data interpretation.
Interpretation
The interpretation “25% of data lies below this value” holds significant importance in the context of finding the lower quartile. It represents the fundamental purpose of calculating the lower quartile and underscores its role in statistical analysis.
By identifying the value below which 25% of the data lies, the lower quartile provides valuable insights into the distribution and variability of data. It serves as a benchmark, dividing the data into four equal parts, and allows researchers and practitioners to make informed decisions based on a comprehensive understanding of the data.
For instance, in a dataset representing the exam scores of a class, the lower quartile would indicate the score below which 25% of the students scored. This information is crucial for educators as it helps them identify areas where students may need additional support or targeted interventions.
Furthermore, the interpretation of the lower quartile has practical applications in various fields. In quality control, it helps manufacturers identify potential defects or non-conformities in their products. In risk assessment, it assists analysts in determining the likelihood and severity of potential risks.
Overall, understanding the interpretation of the lower quartile as “25% of data lies below this value” is essential for accurately calculating this statistical measure and leveraging its insights for informed decision-making.
Lower Quartile Interpretation | |
---|---|
Definition | Identifies the value below which 25% of the data lies. |
Significance | Provides insights into data distribution and variability. |
Applications | Supports decision-making in various fields, including education, quality control, and risk assessment. |
Significance
Unveiling the significance of the lower quartile lies in its ability to provide valuable insights into data distribution and variability. This statistical measure serves as a powerful tool for understanding the spread and central tendency of data, offering a deeper perspective on the underlying patterns and relationships within a dataset.
- Data Distribution: The lower quartile helps identify how data is distributed. By indicating the value below which 25% of the data lies, it reveals whether the data is skewed towards higher or lower values. This understanding aids in making informed decisions and drawing accurate conclusions from the data.
- Data Variability: The lower quartile, in conjunction with other quartiles (such as the upper quartile), provides insights into the variability of data. The distance between the lower and upper quartiles, known as the interquartile range, measures the spread of the middle 50% of the data. This information is crucial for assessing the consistency and reliability of data.
- Outliers Identification: In certain cases, the lower quartile can assist in identifying outliers or extreme values within a dataset. Values that fall significantly below the lower quartile may indicate potential outliers that require further investigation or verification.
- Data Comparison: The lower quartile enables comparisons between different datasets or subgroups within a dataset. By analyzing the lower quartiles of multiple groups, researchers can identify similarities and differences in data distribution and variability, leading to a better understanding of the underlying factors.
In conclusion, the significance of the lower quartile lies in its ability to illuminate data distribution and variability. By providing valuable insights into the spread and central tendency of data, the lower quartile empowers researchers and practitioners to make informed decisions, draw accurate conclusions, and gain a deeper understanding of the patterns and relationships within their data.
Applications
Delving into the applications of the lower quartile unveils its significance in various fields, providing valuable insights for decision-making and analysis. Here’s how the lower quartile finds practical applications in data analysis, quality control, and risk assessment:
-
Data Analysis:
In data analysis, the lower quartile plays a crucial role in understanding data distribution and variability. By identifying the value below which 25% of the data lies, analysts can uncover patterns, trends, and potential outliers. This information aids in making informed decisions, drawing accurate conclusions, and gaining a comprehensive overview of the data. -
Quality Control:
In quality control processes, the lower quartile serves as a benchmark for assessing product quality. By setting the lower quartile as a threshold, manufacturers can identify products that fall below a certain quality level. This enables proactive measures to maintain high standards, reduce defects, and ensure customer satisfaction. -
Risk Assessment:
In risk assessment, the lower quartile helps quantify and evaluate potential risks. By analyzing the lower quartile of historical data, analysts can estimate the likelihood and severity of future risks. This information supports informed decision-making, risk mitigation strategies, and resource allocation for risk management.
In summary, the lower quartile finds practical applications in diverse fields, empowering professionals to analyze data effectively, maintain quality standards, and assess risks accurately. Understanding how to find the lower quartile is essential for unlocking these valuable applications and leveraging data-driven insights for better decision-making.
Median Relationship
Establishing a connection between the median and lower quartile is crucial for understanding the concept of the lower quartile and its significance in data analysis. The median, representing the middle value of a dataset when assorted in numerical order, serves as a reference point for the lower quartile.
-
Understanding the Relationship:
The lower quartile, as defined earlier, represents the value below which 25% of the data lies. Since the median represents the middle value, it logically follows that the lower quartile must be below the median. This relationship holds true regardless of the size or distribution of the dataset. -
Visualizing the Relationship:
Consider a dataset represented graphically. The median divides the dataset into two equal halves, with 50% of the data falling below the median and 50% above it. The lower quartile, on the other hand, marks the boundary between the bottom 25% and the top 75% of the data. Therefore, the lower quartile is always positioned to the left of the median on the number line. -
Example:
To illustrate this relationship, let’s consider a dataset of exam scores: {40, 55, 60, 70, 80, 90, 100}. The median of this dataset is 70, as it lies in the middle when assorted in numerical order. The lower quartile, on the other hand, is 55, as it separates the bottom 25% of the data (scores below 55) from the top 75%.
In summary, understanding the relationship between the lower quartile and median is essential for accurately finding the lower quartile. The lower quartile is always below the median, and this relationship provides valuable insights into the distribution of data and its central tendencies.
Interquartile Range
Unveiling the connection between “Interquartile Range: Distance between lower and upper quartiles” and “how to find the lower quartile” is crucial for comprehensive data analysis. The interquartile range (IQR) plays a pivotal role in understanding the spread and variability of data, providing valuable insights that complement the lower quartile.
The interquartile range is calculated as the difference between the upper quartile (Q3) and the lower quartile (Q1): IQR = Q3 – Q1. It represents the range of values that encompasses the middle 50% of the data, excluding potential outliers.
In the context of finding the lower quartile, the interquartile range provides essential information about the distribution of data below the median. A smaller IQR indicates that the data is tightly clustered around the median, while a larger IQR suggests greater variability in the data.
For example, consider two datasets with the same lower quartile of 25. Dataset A has an IQR of 10, indicating that the middle 50% of the data falls within a relatively narrow range. In contrast, Dataset B has an IQR of 20, suggesting that the data is more spread out and variable.
Understanding the interquartile range in conjunction with the lower quartile enables researchers and practitioners to make informed decisions and draw accurate conclusions from their data. By considering the spread and variability of data, they can identify patterns, trends, and potential outliers, leading to a more comprehensive analysis.
In summary, the interquartile range is an essential component of finding the lower quartile, providing insights into the distribution and variability of data. Understanding this connection empowers data analysts to make informed decisions and gain a deeper understanding of their data.
Example
This example elucidates the practical significance of the lower quartile in the context of educational assessment. By identifying the score below which 25% of students scored, educators can gain valuable insights into student performance and make informed decisions about teaching strategies and interventions.
-
Understanding Student Performance:
The lower quartile provides a benchmark for evaluating student achievement. By comparing the lower quartile to the overall class average or other relevant metrics, educators can assess the distribution of grades and identify areas where students may need additional support. -
Targeted Interventions:
The lower quartile can help educators target interventions effectively. By focusing on students who scored below the lower quartile, educators can provide tailored support and resources to help them improve their academic performance. -
Grading and Assessment:
The lower quartile can inform grading practices and assessment design. By considering the spread of scores and the lower quartile, educators can create assessments that are fair, challenging, and reflective of student learning. -
Comparative Analysis:
The lower quartile enables comparative analysis across different student groups or cohorts. By comparing the lower quartiles of various subgroups, educators can identify disparities in performance and develop strategies to address equity gaps.
In summary, the example of the lower quartile in the context of exam scores underscores its practical utility in educational settings. By providing insights into student performance, guiding targeted interventions, informing grading practices, and enabling comparative analysis, the lower quartile empowers educators to make informed decisions and improve student outcomes.
Relevance
Delving into the relevance of the lower quartile, we uncover its significance in understanding data distribution and patterns. Finding the lower quartile is not merely a mathematical exercise; it empowers us with crucial information that aids in informed decision-making and data-driven analysis.
-
Data Distribution:
The lower quartile serves as a pivotal indicator of how data is distributed. By identifying the value below which 25% of the data lies, we gain insights into whether the data is skewed towards higher or lower values. This understanding helps in identifying potential outliers, understanding the central tendency, and making informed conclusions about the data. -
Variability Assessment:
In conjunction with other quartiles, the lower quartile provides valuable information about the variability of data. The interquartile range, calculated as the difference between the upper and lower quartiles, measures the spread of the middle 50% of the data. This assessment helps in understanding the consistency and reliability of the data, and in identifying potential patterns or trends. -
Data Comparisons:
The lower quartile enables effective comparisons between different datasets or subgroups within a dataset. By analyzing the lower quartiles of multiple groups, researchers can identify similarities and differences in data distribution and variability. This comparative analysis helps in understanding group dynamics, identifying disparities, and making informed decisions based on data-driven insights. -
Outlier Detection:
In certain cases, the lower quartile can assist in identifying outliers or extreme values within a dataset. Values that fall significantly below the lower quartile may indicate potential outliers that require further investigation or verification. This detection process helps in ensuring data integrity, improving the reliability of analysis, and making informed decisions.
In summary, understanding the relevance of the lower quartile is crucial for finding the lower quartile and leveraging its insights for informed decision-making. The lower quartile provides valuable information about data distribution, variability, and patterns, empowering researchers and analysts to make the most of their data and draw accurate conclusions.
Frequently Asked Questions on Finding the Lower Quartile
To enhance your understanding of the lower quartile, we have compiled a list of frequently asked questions and their respective answers. This FAQ section will address common concerns and misconceptions, providing you with a comprehensive overview of this statistical measure.
Question 1: What is the significance of the lower quartile?
The lower quartile holds significant importance as it provides valuable insights into the distribution and variability of data. It helps identify the value below which 25% of the data lies, offering a benchmark for understanding the central tendency and spread of data.
Question 2: How do I find the lower quartile of a dataset?
To find the lower quartile, follow these steps: order the data from smallest to largest, identify the position of the lower quartile using the formula (n+1)/4, and locate the value at that position in the ordered dataset.
Question 3: What is the relationship between the lower quartile and the median?
The lower quartile is always below the median. The median represents the middle value of a dataset, while the lower quartile marks the boundary between the bottom 25% and top 75% of data.
Question 4: How can I interpret the lower quartile in a practical context?
In a dataset representing exam scores, the lower quartile indicates the score below which 25% of students scored. This information aids educators in identifying areas where students may require additional support or targeted interventions.
Question 5: What is the interquartile range, and how is it related to the lower quartile?
The interquartile range (IQR) measures the spread of the middle 50% of data and is calculated by subtracting the lower quartile from the upper quartile. It provides insights into the variability and consistency of data.
Question 6: Why is it important to consider the lower quartile in data analysis?
The lower quartile, along with other quartiles, helps in understanding data distribution, identifying outliers, and making informed decisions based on data. It provides a comprehensive view of data, enabling researchers and analysts to draw accurate conclusions and make meaningful comparisons.
In summary, the lower quartile is a crucial statistical measure that provides valuable insights into data distribution and variability. Understanding how to find and interpret the lower quartile empowers researchers, analysts, and professionals to make informed decisions and gain a deeper understanding of their data.
Stay tuned for our next article, where we delve into advanced concepts related to the lower quartile and its applications in various fields.
Tips on Finding the Lower Quartile
Unveiling the lower quartile requires a systematic approach and an understanding of its significance. Here are some valuable tips to guide you in finding the lower quartile accurately and effectively:
Tip 1: Order Your Data
Begin by arranging your data in ascending order, from the smallest to the largest value. This organized arrangement is essential for identifying the position of the lower quartile within the dataset.
Tip 2: Determine the Position
To find the position of the lower quartile, use the formula: (n+1)/4, where ‘n’ represents the total number of data points in your dataset. This calculation will give you the position of the lower quartile in the ordered dataset.
Tip 3: Locate the Value
Once you have determined the position of the lower quartile, locate the value at that position in your ordered dataset. This value represents the lower quartile, below which 25% of your data lies.
Tip 4: Consider the Median Relationship
Remember that the lower quartile is always below the median, which represents the middle value of your dataset. This relationship provides valuable insights into the distribution of your data.
Tip 5: Use the Interquartile Range
The interquartile range, calculated as the difference between the upper quartile and the lower quartile, measures the spread of the middle 50% of your data. Understanding the interquartile range complements the information provided by the lower quartile.
Summary:
By following these tips, you can accurately find the lower quartile and leverage its insights to understand your data distribution better. This statistical measure provides a foundation for further analysis and informed decision-making.
Finding the Lower Quartile
This comprehensive guide has delved into the intricacies of “how to find the lower quartile,” providing a thorough understanding of this valuable statistical measure. We have explored its definition, formula, interpretation, significance, applications, and relevance, equipping you with the knowledge to confidently find and interpret the lower quartile in your own datasets.
Unveiling the lower quartile unveils crucial insights into data distribution and variability. By understanding how to find this statistical measure, you can uncover patterns, identify trends, and make informed decisions based on data-driven analysis. Whether you are a researcher, analyst, or professional, mastering the lower quartile empowers you to gain a deeper understanding of your data and make a meaningful impact in various fields.
Youtube Video:
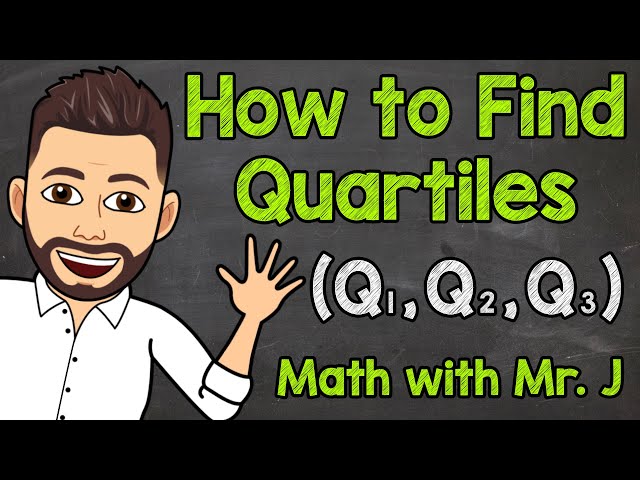